You are looking for information, articles, knowledge about the topic nail salons open on sunday near me apriori 알고리즘 파이썬 on Google, you do not find the information you need! Here are the best content compiled and compiled by the https://chewathai27.com/to team, along with other related topics such as: apriori 알고리즘 파이썬 Apriori 알고리즘 구현, 파이썬 데이터 분석 알고리즘, 아프리오리 알고리즘, Apriori 알고리즘, Apriori leverage conviction, Association 분석, 장바구니 분석 SQL, A priori 파이썬
Table of Contents
[데이터분석] 장바구니 분석(apriori 알고리즘) 사용 및 해석하기
- Article author: lemontia.tistory.com
- Reviews from users: 15986
Ratings
- Top rated: 4.8
- Lowest rated: 1
- Summary of article content: Articles about [데이터분석] 장바구니 분석(apriori 알고리즘) 사용 및 해석하기 apriori 알고리즘을 사용하기 위해서는 다음과 같은 구조로 데이터셋이 되어야 한다. dataset … 파이썬 Apriori 알고리즘(장바구니분석, 연관분석). …
- Most searched keywords: Whether you are looking for [데이터분석] 장바구니 분석(apriori 알고리즘) 사용 및 해석하기 apriori 알고리즘을 사용하기 위해서는 다음과 같은 구조로 데이터셋이 되어야 한다. dataset … 파이썬 Apriori 알고리즘(장바구니분석, 연관분석). apriori 알고리즘을 사용하기 위해서는 다음과 같은 구조로 데이터셋이 되어야 한다. dataset = [ [‘아메리카노’, ‘카페라떼’], [‘카페라떼’, ‘아메리카노’, ‘카푸치노’], [‘바닐라라떼’, ‘아메리카노’], … ] DB..
- Table of Contents:
관련글
댓글2
공지사항
최근글
인기글
최근댓글
태그
전체 방문자
티스토리툴바
![[데이터분석] 장바구니 분석(apriori 알고리즘) 사용 및 해석하기](https://img1.daumcdn.net/thumb/R800x0/?scode=mtistory2&fname=https%3A%2F%2Fblog.kakaocdn.net%2Fdn%2FcgdUOK%2Fbtqz9tnpQNM%2Fj7nwcJkLUbizYxJQfymYe1%2Fimg.png)
파이썬 Apriori 알고리즘(장바구니분석, 연관분석) : 네이버 블로그
- Article author: m.blog.naver.com
- Reviews from users: 41032
Ratings
- Top rated: 4.3
- Lowest rated: 1
- Summary of article content: Articles about 파이썬 Apriori 알고리즘(장바구니분석, 연관분석) : 네이버 블로그 파이썬 Apriori 알고리즘(장바구니분석, 연관분석) … 지지도를 0.5로 놓고 apriori를 돌려보면 아래와 같이 결과가 출력된다. …
- Most searched keywords: Whether you are looking for 파이썬 Apriori 알고리즘(장바구니분석, 연관분석) : 네이버 블로그 파이썬 Apriori 알고리즘(장바구니분석, 연관분석) … 지지도를 0.5로 놓고 apriori를 돌려보면 아래와 같이 결과가 출력된다.
- Table of Contents:
카테고리 이동
Le Nuit
이 블로그
Python
카테고리 글
카테고리
이 블로그
Python
카테고리 글
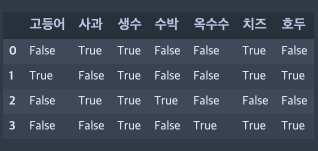
[python] 연관규칙분석(ASSOCIATION RULE ANALYSIS)
- Article author: hezzong.tistory.com
- Reviews from users: 10926
Ratings
- Top rated: 3.0
- Lowest rated: 1
- Summary of article content: Articles about [python] 연관규칙분석(ASSOCIATION RULE ANALYSIS) Apiori 알고리즘이 널리 쓰이는 이유는 알고리즘 구현이 비교적 간단하고 높은 수준의 성능을 보이기 때문입니다. 1. Apriori algorithm. 2. FP-Growth … …
- Most searched keywords: Whether you are looking for [python] 연관규칙분석(ASSOCIATION RULE ANALYSIS) Apiori 알고리즘이 널리 쓰이는 이유는 알고리즘 구현이 비교적 간단하고 높은 수준의 성능을 보이기 때문입니다. 1. Apriori algorithm. 2. FP-Growth … 연관규칙분석이란? 연관 규칙 분석이란 어떤 두 아이템 집합이 번번히 발생하는가를 알려주는 일련의 규칙들을 생성하는 알고리즘입니다. 경영학에서 장바구니 분석(Market Basket Analysis)으로 알려진 이 알고..
- Table of Contents:
관련글
댓글2
공지사항
최근글
인기글
최근댓글
태그
전체 방문자
![[python] 연관규칙분석(ASSOCIATION RULE ANALYSIS)](https://img1.daumcdn.net/thumb/R800x0/?scode=mtistory2&fname=https%3A%2F%2Fblog.kakaocdn.net%2Fdn%2FbDzfBM%2FbtqBXU5ueHg%2F8y7c2Lq0k6qEenuH8ZUUbK%2Fimg.png)
The Apriori algorithm: what it is and how to use it in Python
- Article author: hands-on.cloud
- Reviews from users: 36022
Ratings
- Top rated: 3.7
- Lowest rated: 1
- Summary of article content: Articles about The Apriori algorithm: what it is and how to use it in Python The Apriori algorithm is a well-known Machine Learning algorithm that is used for association rule learning. association rule learning is a … …
- Most searched keywords: Whether you are looking for The Apriori algorithm: what it is and how to use it in Python The Apriori algorithm is a well-known Machine Learning algorithm that is used for association rule learning. association rule learning is a … This article covered the Apriori algorithm theory and its Python programming language implementation.
- Table of Contents:
Table of contents
Algorithm Overview
Using Apriori algorithm in Python
Summary
Related articles
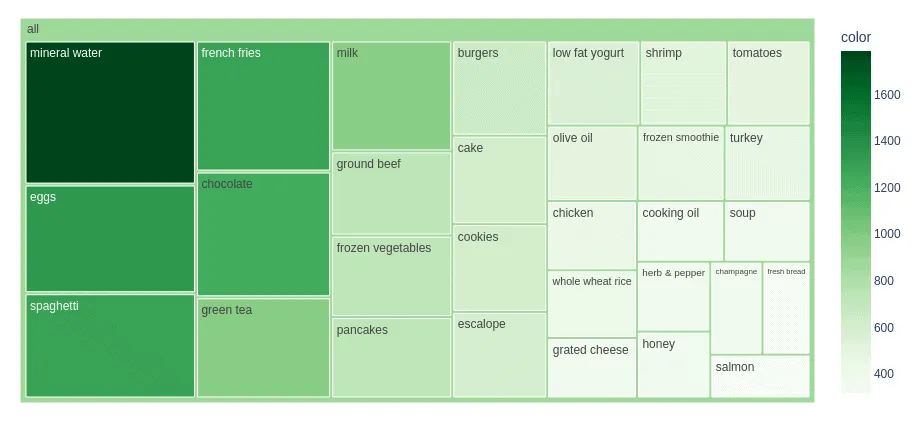
[Python] Apriori algorithm:: 연관규칙분석 (1)
- Article author: ordo.tistory.com
- Reviews from users: 46143
Ratings
- Top rated: 3.5
- Lowest rated: 1
- Summary of article content: Articles about [Python] Apriori algorithm:: 연관규칙분석 (1) [Python] Apriori algorithm:: 연관규칙분석 (1). JKyun 2018. 7. 18. 16:26. 안녕하세요. 우주신 입니다. 이번 포스팅에서는 연관규칙 알고리즘 중 가장 먼저 접하게 … …
- Most searched keywords: Whether you are looking for [Python] Apriori algorithm:: 연관규칙분석 (1) [Python] Apriori algorithm:: 연관규칙분석 (1). JKyun 2018. 7. 18. 16:26. 안녕하세요. 우주신 입니다. 이번 포스팅에서는 연관규칙 알고리즘 중 가장 먼저 접하게 … 안녕하세요. 우주신 입니다. 이번 포스팅에서는 연관규칙 알고리즘 중 가장 먼저 접하게 되는 Apriori 알고리즘에 대해 알아보겠습니다. Apriori 알고리즘은 빈발항목집합(frequent itemsets) 및 연관규칙분석을..
- Table of Contents:
태그
관련글
댓글3
공지사항
최근글
인기글
최근댓글
태그
전체 방문자
Data Science Apriori Algorithm in Python – Market Basket Analysis
- Article author: intellipaat.com
- Reviews from users: 33795
Ratings
- Top rated: 4.5
- Lowest rated: 1
- Summary of article content: Articles about Data Science Apriori Algorithm in Python – Market Basket Analysis Apriori algorithm assumes that any subset of a frequent itemset must be frequent. Say, a transaction containing {wine, chips, bread} also contains {wine, bread} … …
- Most searched keywords: Whether you are looking for Data Science Apriori Algorithm in Python – Market Basket Analysis Apriori algorithm assumes that any subset of a frequent itemset must be frequent. Say, a transaction containing {wine, chips, bread} also contains {wine, bread} … Data Science Apriori algorithm is a data mining technique that is used for Association Rule Mining. Learn how to use python in Association Rule Mining and Apriori algorithm.
- Table of Contents:
Introduction to Apriori Algorithm in Python
What Is Association Rule Mining
What Is an Apriori Algorithm
How Does the Apriori Algorithm Work
Hands-on Apriori Algorithm in Python- Market Basket Analysis
Limitations of Apriori Algorithm
Improvements
Applications of Apriori Algorithm
What Did We Learn
Related Articles
Associated Courses
All Tutorials
Subscribe to our newsletter
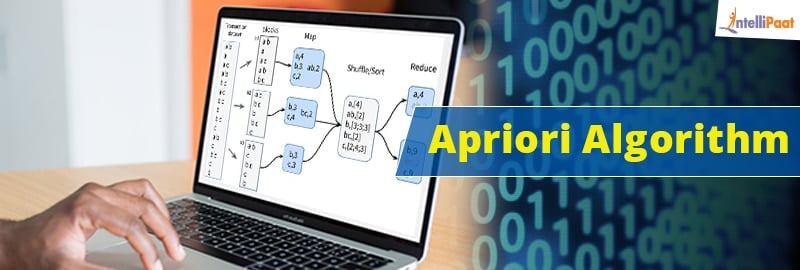
8. 연관 규칙 분석(Association Rule Analysis) with Python
- Article author: zephyrus1111.tistory.com
- Reviews from users: 47142
Ratings
- Top rated: 3.5
- Lowest rated: 1
- Summary of article content: Articles about 8. 연관 규칙 분석(Association Rule Analysis) with Python Apriori 알고리즘은 다음과 같이 빈발 품목 집합을 찾는다. 1) 최소 지지도(최소 발생 비율)를 설정한다. p= … …
- Most searched keywords: Whether you are looking for 8. 연관 규칙 분석(Association Rule Analysis) with Python Apriori 알고리즘은 다음과 같이 빈발 품목 집합을 찾는다. 1) 최소 지지도(최소 발생 비율)를 설정한다. p= … 이번 포스팅에서는 데이터 간의 관계를 탐색하기 위한 방법으로 마케팅 분야에서 많이 활용되고 있는 연관 규칙 분석(마케팅에서는 장바구니 분석이라고도 한다) 대해서 알아보고자 한다. 여기서 다루는 내용은 다..
- Table of Contents:
1 연관 규칙 분석이란 무엇인가
2 연관 규칙 분석 방법
3 고려 사항
4 예제 with Python
관련글
댓글0
전체 방문자
최근글
인기글
티스토리툴바
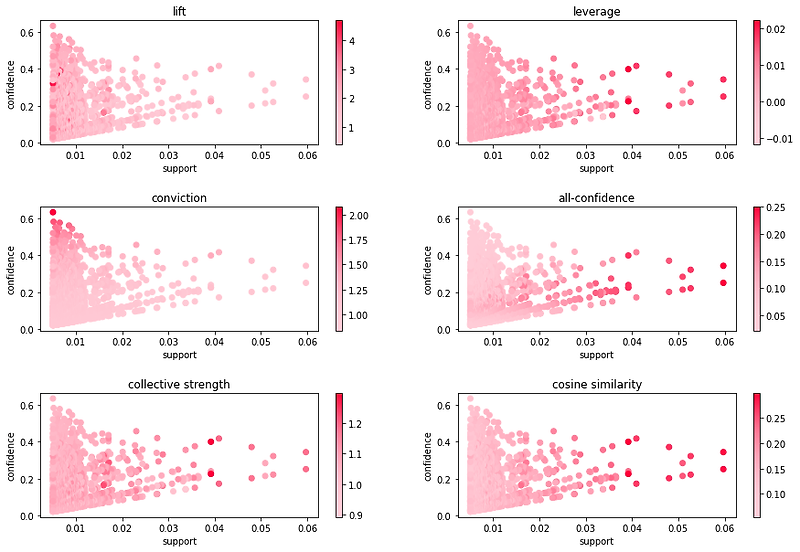
파이썬 코딩으로 말하는 데이터 분석 – 4. 연관 (Apriori 알고리즘)
- Article author: hamait.tistory.com
- Reviews from users: 29971
Ratings
- Top rated: 3.5
- Lowest rated: 1
- Summary of article content: Articles about 파이썬 코딩으로 말하는 데이터 분석 – 4. 연관 (Apriori 알고리즘) 파이썬 코딩으로 말하는 데이터 분석 – 4. 연관 (Apriori 알고리즘). [하마] 이승현 ([email protected]) 2017. 1. 19. 18:58. 요즘 데이터분석과 관련하여 텐서 … …
- Most searched keywords: Whether you are looking for 파이썬 코딩으로 말하는 데이터 분석 – 4. 연관 (Apriori 알고리즘) 파이썬 코딩으로 말하는 데이터 분석 – 4. 연관 (Apriori 알고리즘). [하마] 이승현 ([email protected]) 2017. 1. 19. 18:58. 요즘 데이터분석과 관련하여 텐서 … 요즘 데이터분석과 관련하여 텐서플로우와 스파크(ML)등의 머신러닝 솔루션들이 굉장히 유행하고 있습니다. 물론 저것들이 삶을 편안하게 만들어주기도 하지만 대부분의 데이터 분석은 저런 거창한 것 말고 평..
- Table of Contents:
HAMA 블로그
파이썬 코딩으로 말하는 데이터 분석 – 4 연관 (Apriori 알고리즘) 본문
See more articles in the same category here: https://chewathai27.com/to/blog.
[데이터분석] 장바구니 분석(apriori 알고리즘) 사용 및 해석하기
반응형
apriori 알고리즘을 사용하기 위해서는 다음과 같은 구조로 데이터셋이 되어야 한다.
dataset = [ [‘아메리카노’, ‘카페라떼’], [‘카페라떼’, ‘아메리카노’, ‘카푸치노’], [‘바닐라라떼’, ‘아메리카노’], … ]
DBMS에 이런식으로 데이터가 저장되어 있을리가 만무하다. 전처리를 위해 어떻게 처리하면 좋을지 이전 포스팅에 추가해두었다.
https://blog.naver.com/varkiry05/221724021065
여기서는 샘플을 만들어 진행하고자 한다.
데이터셋 샘플 만들기
dataset = [ [‘아메리카노’, ‘카페라떼’], [‘카페라떼’, ‘아메리카노’, ‘카푸치노’], [‘바닐라라떼’, ‘아메리카노’], [‘녹차라떼’, ‘카페라떼’, ‘아메리카노’], [‘카페모카’, ‘아메리카노’], [‘아메리카노’, ‘카페라떼’], [‘초콜릿’, ‘아메리카노’], [‘아메리카노’], [‘카페모카’, ‘카페라떼’] ]
pandas, mlxtend 패키지를 로드한다
from mlxtend.preprocessing import TransactionEncoder from mlxtend.frequent_patterns import apriori
학습 시작
te = TransactionEncoder() te_result = te.fit(dataset).transform(dataset)
te_result 결과를 보면 다음과 같다.
이것을 보기 좋게 데이터프레임으로 넣는다
데이터를 보면 모든 상품이 컬럼명으로 들어가있고, 각 row마다 포함된 상품에 True 를 반환한다.
인덱스 0(첫번째)줄을 보면 아메리카노와 카페라떼를 구매했기 때문에 두개의 컬럼에만 True 되어있다.
이제 apriori 알고리즘을 사용해보자
itemset = apriori(df, use_colnames=True) itemset
특별히 옵션을 주지 않으면 기본 지지도(support)는 0.5로 설정된다. 이번에는 옵션을 넣어 지지도를 좀더 낮춰본다.
itemset = apriori(df, min_support=0.1, use_colnames=True) itemset
아메리카노를 제외하면 지지도가 대부분 낮다.
실제 데이터를 돌렸을땐 이보다 더 낮은 지지도를 형성하는 경우가 많았다.
심할때는 옵션에 support 를 0.001 을 주기도 했다.
이제 신뢰도를 확인하자
min_threshold 의 기본값은 0.8 이다.
그런데 위에서 지지도가 낮은것을 억지로 보이게 했으니 신뢰도도 상대적으로 낮게 설정해야 목록이 좀 보인다.
from mlxtend.frequent_patterns import association_rules association_rules(itemset, metric=”confidence”, min_threshold=0.1)
min_threshold 를 0.1 로 설정한 경우
min_threshold 를 기본값(0.8)으로 둘 경우
lift(향상도) 수치가 1보다 큰것들이 있는데, 1보다 클수록 우연히 일어나지 않았다는 표시다. 아무런 관계가 없다면 1로 표시된다.
위의 표를 해석해보자면 아메리카노를 구매할 때 초콜릿이나 바닐라라떼, 카페모카 등을 구매하는 경우가 있고 셋의 신뢰도(confidence)가 0.1818로 같다.
– 아메리카노 – 바닐라라떼: 0.1818
– 아메리카노- 초콜릿: 0.1818
– 아메리카노 – 카페모카: 0.1818
이중 바닐라라떼의 향상도가 1보다 커 가장 높은 인기를 가진다는 의미다.
이쯤에서 각 용어들에 대해 조금 알아두면 좋을 듯 하다.
– support(지지도)
전체 거래에서 특정 물품 A와 B가 동시에 거래되는 비중
해당 규칙이 얼마나 의미있는지 보여줌.
지지도 = P(A∩B)
:A와 B가 동시에 일어난 횟수 / 전체 거래 횟수
– confiddence(신뢰도)
A를 포함하는 거래 중 A와 B가 동시에 거래되는 비중
신뢰도 = P(A∩B) / P(A)
:A와 B가 동시에 일어난 횟수 / A가 일어난 횟수
– lift(향상도)
A라는 상품에서 신뢰도가 동일한 상품 B와 C가 존재할 때, 어떤 상품을 더 추천해야 좋을지 판단.
A와 B가 동시에 거래된 비중을 A와 B가 서로 독립된 사건일 때 동시에 거래된 비중으로 나눈 값
향상도 = P(A∩B) / P(A)*P(B) = P (B|A) / P (B)
: A와 B가 동시에 일어난 횟수 / A, B가 독립된 사건일 때 A,B가 동시에 일어날 확률
끝.
참조:
https://needjarvis.tistory.com/59
http://blog.naver.com/PostView.nhn?blogId=eqfq1&logNo=221444712369&parentCategoryNo=&categoryNo=45&viewDate=&isShowPopularPosts=true&from=search
반응형
[python] 연관규칙분석(ASSOCIATION RULE ANALYSIS)
연관규칙분석이란?
연관 규칙 분석이란 어떤 두 아이템 집합이 번번히 발생하는가를 알려주는 일련의 규칙들을 생성하는 알고리즘입니다. 경영학에서 장바구니 분석(Market Basket Analysis)으로 알려진 이 알고리즘은 누구나 한 번쯤 경험해보았을 것입니다. 오늘은 최근 인터넷 쇼핑 및 상품 진열 등 다양한 컨텐츠 기반 추천(contents-based recommendation)에 널리 사용되고 있는 이 연관규칙분석 알고리즘에 대해 알아보고자 합니다.
사실 상품 추천에는 순차분석 (Sequence Analysis), Collaborative Filtering , Contents-based recommendation 등 여러가지 분석 기법이 존재합니다. 그 중 하나가 바로 연관규칙분석입니다. 연관규칙분석은 거래(transaction)와 항목(item)으로 구성되어 있는 경우 분석이 가능합니다.
연관규칙분석, 장바구니분석 (Association Rule Analysis, Market Basket Analysis) : 고객의 대규모 거래데이터로부터 함께 구매가 발생하는 규칙 ( 예 : A à 동시에 B) 을 도출하여 , 고객이 특정 상품 구매 시 이와 연관성 높은 상품을 추천
순차분석 (Sequence Analysis) : 고객의 시간의 흐름에 따른 구매 패턴 (A à 일정 시간 후 B) 을 도출하여 , 고객이 특정 상품 구매 시 일정 시간 후 적시에 상품 추천
Collaborative Filtering : 모든 고객의 상품 구매 이력을 수치화하고 , 추천 대상이 되는 고객 A 와 다른 고객 B 에 대해 상관계수를 비교해서 , 서로 높은 상관이 인정되는 경우 고객 B 가 구입 완료한 상품 중에 고객 A 가 미구입한 상품을 고객 A 에게 추천
Contents-based recommendation : 고객이 과거에 구매했던 상품들의 속성과 유사한 다른 상품 아이템 중 미구매 상품을 추천 ( ↔ Collaborative Filtering 은 유사 고객 을 찾는 것과 비교됨 )
Who-Which modeling : 특정 상품 ( 군 ) 을 추천하는 모형을 개발 ( 예 : 신형 G5 핸드폰 추천 스코어모형 ) 하여 구매 가능성 높은 ( 예 : 스코어 High) 고객 ( 군 ) 대상 상품 추천
연관규칙분석에서 규칙의 효용성
연관규칙분석은 이름에서 의미하는 그대로 규칙 베이스로 합니다. 여기서 예시를 한 번 봅시다.
dataset=[[‘사과’,’치즈’,’생수’], [‘생수’,’호두’,’치즈’,’고등어’], [‘수박’,’사과’,’생수’], [‘생수’,’호두’,’치즈’,’옥수수’]]
위와 같은 데이터 셋이 있을 때,
1 : (사과,치즈.생수)
2: (생수,호두,치즈,고등어)
3: (수박,사과,생수)
4: (생수,호두,치즈,옥수수)
규칙
– 사과를 산 사람은 생수를 산다.
– 생수를 산 사람은 고등어를 산다.
– 치즈를 산 사람 생수를 산다.
…
등등 엄청나게 많은 규칙들을 생성할 수 있습니다. 그렇다면 어떤 규칙이 좋은 규칙인지 어떻게 판단할 수 있을까요?
좋은 규칙을 판단하는 세가지 지표가 있습니다.
지지도(support) : 한 거래 항목 안에 A와 B를 동시에 포함하는 거래의 비율. 지지도는 A와 B가 함께 등장할 확률 이다. 전체 거래의 수를 A와 B가 동시에 포함된 거래수를 나눠주면 구할 수 있다.
신뢰도(confidence) : 항목 A가 포함하는 거래에 A와 B가 같이포함될 확률. 신뢰도는 조건부 확률과 유사하다. A가 일어났을 때 B의 확률 이다. A의 확률을 A와 B가 동시에 포함될 확률을 나눠주면 구할 수 있다.
A가 일어났을 때 B의 확률 향상도(lift) : A가 주어지지 않을 때의 품목 B의 확률에 비해 A가 주어졌을 때 품목 B의 증가 비율. B의 확률이 A가 일어났을 때 B의 확률을 나눴을 때 구할 수 있다. lift 값은 1이면 서로 독립적인 관계 이며 1보다 크면 두 품목이 서로 양의 상관관계, 1보다 작으면 두 품목이 서로 음의 상관관계 이다. A와 B가 독립이면 분모, 분자가 같기 때문에 1이 나온다.
연관규칙분석 알고리즘
연관규칙분석의 대표적인 알고리즘은 3가지로 분류할 수 있습니다. 하지만 저는 가장 많이 쓰이고 예제도 많은 Apiori 알고리즘에 대해서만 다루려고 합니다. Apiori 알고리즘이 널리 쓰이는 이유는 알고리즘 구현이 비교적 간단하고 높은 수준의 성능을 보이기 때문입니다.
1. Apriori algorithm
2. FP-Growth algorithm
3. DHP algorithm
Example) 장바구니 분석
사실 지금까지는 Apriori 알고리즘을 왜 사용해야하는지 그 개념이 무엇인지를 알기 위한 과정이였습니다. 대략적인 과정을 알았으니 간단한 예제를 통해 어떻게 활용할 수 있을지 알아봅시다.
1. 필요한 모듈 import
import pandas as pd from mlxtend.preprocessing import TransactionEncoder from mlxtend.frequent_patterns import apriori, association_rules
– pandas : 파이썬에서 사용하는 데이터분석 라이브러리로, 행과 열로 이루어진 데이터 객체를 만들어 다룰 수 있게 되며 보다 안정적으로 대용량의 데이터들을 처리하는데 매우 편리한 도구
– mlxtend : 일상적인 데이터 사이언스 작업에 유용한 도구들로 구성된 파이썬 라이브러리
2. 데이터 셋 생성 및 가공
dataset = [[‘Milk’, ‘Onion’, ‘Nutmeg’, ‘Eggs’, ‘Yogurt’], [‘Onion’, ‘Nutmeg’, ‘Eggs’, ‘Yogurt’], [‘Milk’, ‘Apple’, ‘Eggs’], [‘Milk’, ‘Unicorn’, ‘Corn’, ‘Yogurt’], [‘Corn’, ‘Onion’, ‘Onion’, ‘Ice cream’, ‘Eggs’]] te = TransactionEncoder() te_ary = te.fit(dataset).transform(dataset) df = pd.DataFrame(te_ary, columns=te.columns_)
데이터 셋 생성과정은 문제 없이 이해할 수있을 것 입니다. 마지막 두 줄에 대해서만 따로 알아봅시다.
주어진 코드에서 fit 함수를 통해 dataset은 고유한 라벨을 갖게 되고 , transform함수를 통해서 파이썬 리스트를 one-hot 인코딩 된 numPy 배열로 변환합니다. one-hot 인코딩에 대한 자세한 설명을 원하시면 다음 글을 참고해 주시기 바랍니다. ( https://teddylee777.github.io/machine-learning/python-numpy%EB%A1%9C-one-hot-encoding-%EC%89%BD%EA%B2%8C%ED%95%98%EA%B8%B0 )
배열을 출력해 보면 다음과 같이 확인 할 수 있습니다. 즉, 이 과정을 거치면 (Apple, Corn, Eggs, Ice cream, Milk, Nutmeg, Onion, Unicorn, Yogurt) 을 컬럼으로 갖고 아이템의 유무에 따라 True, false로 표현 된 배열을 갖게 됩니다.
3. Apriori 알고리즘 활용
frequent_itemsets = apriori(df, min_support=0.5, use_colnames=True)
우리는 Apriori 알고리즘을 활용하여 지지도가 0.5 이상인 것들을 알아낼 수있습니다.
지지도가 0.5 이상인 항목
dataset =
[[ ‘Milk’ , ‘Onion’ , ‘Nutmeg’ , ‘Eggs’ , ‘Yogurt’ ] , [ ‘Onion’ , ‘Nutmeg’ , ‘Eggs’ , ‘Yogurt’ ] , [ ‘Milk’ , ‘Apple’ , ‘Eggs’ ] , [ ‘Milk’ , ‘Unicorn’ , ‘Corn’ , ‘Yogurt’ ] , [ ‘Corn’ , ‘Onion’ , ‘Onion’ , ‘Ice cream’ , ‘Eggs’ ]]즉, 5개의 장바구니 중에 Eggs는 4개에 들어가 있으므로 80%의 확률을 갖는다고 할 수 있습니다.
association_rules(frequent_itemsets, metric=”lift”, min_threshold=1)
association_rules 함수를 이용하여 지지도가 0.5가 넘는 항목에 대해 향상도가 양의 상관관계에 있는 것이 무엇인지 알아봅시다.
여기서는 egg와 Onion이 양의 상관관계가 있는 것으로 확인 되네요.
Reference
– https://rfriend.tistory.com/190
– https://ratsgo.github.io/machine%20learning/2017/04/08/apriori/
– https://ordo.tistory.com/89?category=751589
– http://www.birc.co.kr/2017/01/05/%EC%97%B0%EA%B4%80%EC%84%B1-%EB%B6%84%EC%84%9D%EC%9E%A5%EB%B0%94%EA%B5%AC%EB%8B%88-%EB%B6%84%EC%84%9D-%EC%88%9C%EC%B0%A8-%EC%97%B0%EA%B4%80%EC%84%B1-%EB%B6%84%EC%84%9D/
– https://blog.naver.com/eqfq1/221444712369
– https://dbdp.tistory.com/58
– https://jfun.tistory.com/104
– https://doorbw.tistory.com/172
-http://rasbt.github.io/mlxtend/
The Apriori algorithm: what it is and how to use it in Python
The Apriori algorithm is a well-known Machine Learning algorithm that is used for association rule learning. association rule learning is a process of taking a dataset and finding relationships between items in the data. For example, if you have a dataset of grocery store items, you could use association rule learning to find items that are often purchased together. The Apriori algorithm is particularly well-suited for finding association rules in large datasets. It works by first identifying the frequent itemsets in the dataset and then using these itemsets to generate association rules. The Apriori algorithm has been widely used in retail applications such as market basket analysis. It has also been used in other domains such as medicine and biology. This article will cover the Apriori algorithm basics and demonstrate how to use it in Python.
Best Machine Learning Books for Beginners and Experts As Machine Learning becomes more and more widespread, it’s important for both beginners and experts to stay up to date on the latest advancements. For beginners, checking out the best Machine Learning books can help to get a solid understanding of the basics. For experts, reading these books can help to keep pace with the ever-changing landscape. In either case, there are a few key reasons why checking out these books can be beneficial. First, they provide a comprehensive overview of the subject matter. Second, they offer insights from leading experts in the field. And third, they offer concrete advice on how to apply machine learning concepts in real-world scenarios. As machine learning continues to evolve, there’s no doubt that these books will continue to be essential resources for anyone looking to stay ahead of the curve.
Algorithm Overview
The Apriori algorithm is used on frequent itemsets to generate association rules, and it is designed to work on the databases that contain transactions. The process of generating association rules is called association rule mining or association rule learning. We can use these association rules to measure how strong or weak two objects from the dataset are related. Frequent itemsets are those whose support value (see below) is greater than the user-specified minimum support value.
The most common problems that this algorithm helps to solve are:
Product recommendation
Market basket recommendation
There are three major parts of the Apriori algorithm.
Support
Confidence
Lift
Let’s take a deeper look at each one of them.
Let’s imagine we have a history of 3000 customers’ transactions in our database, and we have to calculate the Support, Confidence, and Lift to figure out how likely the customers who’re buying Biscuits will buy Chocolate.
Here are some numbers from our dataset:
3000 customers’ transactions
400 out of 3000 transactions contain Biscuit purchases
600 out of 3000 transactions contain Chocolate purchases
200 out of 3000 transactions describe purchases when customers bought Biscuits and Chocolates together
Support
The Support of an item is defined as the percentage of transactions in which an item appears. In other words, support represents how often an item appears in a transaction. The Apriori algorithm uses a “bottom-up” approach, where it starts with individual items and then finds combinations of items that appear together frequently. The Support threshold is a parameter that is used to determine which item sets are considered frequent. Itemsets that have the Support greater than or equal to the Support threshold are considered frequent.
Item support can be calculated by finding the number of transactions containing a particular item divided by the total number of transactions:
In our case, the support value for biscuits will be:
Confidence
Confidence is a statistical measure used in association rule learning that quantifies the likelihood that an association rule is correct. In other words, confidence measures the reliability of an association rule. The confidence of a rule is calculated as the ratio of the number of times the rule is found to be true to the total number of times it is checked. For example, if a rule has confidence of 80%, this means that out of 100 times the rule is checked, it will be true 80 times.
There are several ways to increase the confidence of a rule, including increasing the Support or decreasing the number of exceptions. However, confidence is not a perfect measure, and it can sometimes lead to overfitting if rules with high confidence are given too much weight. Therefore, it is important to use confidence in conjunction with other measures, such as Support, to ensure that association rules are reliable.
Here’s a mathematical expression to describe how often items in a transaction B appear in transactions containing A:
In our example, the confidence value shows the probability of the fact that customers buy Chocolate if they bought Biscuits. To calculate this value, we need to divide the number of transactions that contain Biscuits and Chocolates by the total number of transactions having Biscuits:
It means we are confident that 50 percent of customers who bought Biscuits will buy Chocolates too.
Lift
The Lift metric is often used to measure the strength of association between two items. Lift is simply the ratio of the observed frequency of two items being bought together to the expected frequency of them being bought together if they were independent. In other words, Lift measures how much more likely two items are to be bought together than would be expected if they were unrelated. A high Lift value indicates a strong association between two items, while a low Lift value indicates a weak association. The Apriori algorithm is designed to find itemsets with a high Lift value.
Lift describes how much confident we are if B will be purchased too when the customer buys A:
In our example, the Lift value shows the potential increase in the ratio of the sale of Chocolates when you sell Biscuits. The larger the value of the lift, the better:
Algorithm steps
The algorithm consists of the following are the steps:
Start with itemsets containing just a single item (Individual items)
Determine the support for itemsets
Keep the itemsets that meet the minimum support threshold and remove itemsets that do not support minimum support
Using the itemsets that are kept from Step 1, generate all the possible itemset combinations.
Repeat steps 1 and 2 until there are no more new itemsets.
Let’s take a look at these steps while using a sample dataset:
First, the algorithm will create a table containing each item set’s support count in the given dataset – the Candidate set:
Let’s assume that we’ve set up the minimum support value to 3, which means the algorithm will drop all the items having a support value of less than three.
The algorithm will take out all the itemsets with a greater support count than the minimum support (frequent itemset) in the next step:
Next, the algorithm will generate the second candidate set (C2) with the help of the frequent itemset (L1) from the previous calculation. Candidate set 2 (C2) will be formed by creating the pairs of itemsets of L1. After creating new subsets, the algorithm will again find the support count from the main transaction table of datasets by calculating how many times these pairs have occurred together in the given dataset.
After that, the algorithm will compare the C2’s support count values with the minimum support count (3), and the itemset with less support count will be eliminated from table C2.
Finally, the algorithm can mine different association rules using the last frequent itemset.
Algorithm disadvantages
While the Apriori algorithm is a powerful tool for finding association rules in large datasets, it has a number of disadvantages that should be considered before using it
First, the algorithm requires a large amount of memory to store all of the possible item sets.
Second, it can be time-consuming to generate all of the possible item sets, especially if the dataset is very large.
Third, the algorithm can sometimes produce false positives, which means that it may identify association rules that do not actually exist.
Finally, the algorithm may not be able to find all of the interesting associations in a dataset if the support and confidence thresholds are set too high.
Despite these disadvantages, this algorithm is still a widely used method for finding association rules and is often applied successfully to large datasets.
Algorithm alternatives
There are several alternatives to the Apriori algorithm for finding frequent itemsets in a dataset. One popular option is the Eclat algorithm, which uses an efficient depth-first search strategy to find itemsets that are close together in the data. Another common alternative is the FP-growth algorithm, which uses a compression technique to represent the data in a more compact form. This can be used to speed up the search for frequent itemsets. Finally, the frequent pattern mining (FPM) method is a general approach that can be used with any of a variety of algorithms, including Apriori. FPM first converts the data into a lattice structure, which is then mined for frequent itemsets using one of several algorithms. Each of these methods has its own advantages and disadvantages, and there is no clear consensus on which one is best. Ultimately, the choice of algorithm will depend on the specific application and dataset.
Using Apriori algorithm in Python
We will use the market basket optimization dataset (you can download the dataset here).
Before starting using the algorithm in Python, we need to install the required modules by running the following commands in the cell of the Jupyter notebook:
%pip install pandas %pip install numpy %pip install plotly %pip install networkx %pip install matplotlib
Exploring dataset
First, let’s import the dataset and get familiar with it. We will use the Pandas DataFrame to store and manipulate our dataset:
# importing module import pandas as pd # dataset data = pd.read_csv(“Market_Basket_Optimisation.csv”) # printing the shape of the dataset data.shape
Output:
The output shows that our dataset contains 7500 rows/observations and 20 columns/attributes. We can print out the first few rows and columns of the dataset to see what kind of data our dataset contains.
# printing the heading data.head()
Output:
Visualizing the dataset
As you can see, there are a lot of null values in our dataset, and it isn’t easy to figure out which item has been purchased more. We can iterate through our data and store each item in a separate NumPy array.
Let’s print out the top 10 most frequent items from the dataset.
# importing module import numpy as np # Gather All Items of Each Transactions into Numpy Array transaction = [] for i in range(0, data.shape[0]): for j in range(0, data.shape[1]): transaction.append(data.values[i,j]) # converting to numpy array transaction = np.array(transaction) # Transform Them a Pandas DataFrame df = pd.DataFrame(transaction, columns=[“items”]) # Put 1 to Each Item For Making Countable Table, to be able to perform Group By df[“incident_count”] = 1 # Delete NaN Items from Dataset indexNames = df[df[‘items’] == “nan” ].index df.drop(indexNames , inplace=True) # Making a New Appropriate Pandas DataFrame for Visualizations df_table = df.groupby(“items”).sum().sort_values(“incident_count”, ascending=False).reset_index() # Initial Visualizations df_table.head(10).style.background_gradient(cmap=’Greens’)
Output:
The output shows that mineral water has been purchased more frequently than other products.
A treemapping is a method for displaying hierarchical data using nested figures, usually rectangles. We can use a treemap to visualize all the items from our dataset more interactive.
# importing required module import plotly.express as px # to have a same origin df_table[“all”] = “all” # creating tree map using plotly fig = px.treemap(df_table.head(30), path=[‘all’, “items”], values=’incident_count’, color=df_table[“incident_count”].head(30), hover_data=[‘items’], color_continuous_scale=’Greens’, ) # ploting the treemap fig.show()
Output:
Data pre-processing
Before getting the most frequent itemsets, we need to transform our dataset into a True – False matrix where rows are transactions and columns are products.
Possible cell values are:
True – the transaction contains the item
– the transaction contains the item False – transaction does not contain the item
# importing the required module from mlxtend.preprocessing import TransactionEncoder # initializing the transactionEncoder te = TransactionEncoder() te_ary = te.fit(transaction).transform(transaction) dataset = pd.DataFrame(te_ary, columns=te.columns_) # dataset after encoded dataset
Output:
We have 121 columns/features at the moment. Extracting the most frequent itemsets from 121 features would be compelling. So, we will start with the Top 50 items.
# select top 50 items first50 = df_table[“items”].head(50).values # Extract Top50 dataset = dataset.loc[:,first50] # shape of the dataset dataset.shape
Output:
Notice that the number of columns is now 50.
Using Apriori algorithm
Now we can use mlxtend module, which contains the Apriori algorithm implementation to get some insights from our data.
# importing the required module from mlxtend.frequent_patterns import apriori, association_rules # Extracting the most frequest itemsets via Mlxtend. # The length column has been added to increase ease of filtering. frequent_itemsets = apriori(dataset, min_support=0.01, use_colnames=True) frequent_itemsets[‘length’] = frequent_itemsets[‘itemsets’].apply(lambda x: len(x)) # printing the frequent itemset frequent_itemsets
Output:
The output shows that mineral water is the most frequently occurring item in our dataset. We can explore the frequent item more to get the inside. For example, we can print out all items with a length of 2, and the minimum support is more than 0.05.
# printing the frequntly items frequent_itemsets[ (frequent_itemsets[‘length’] == 2) & (frequent_itemsets[‘support’] >= 0.05) ]
Output:
The output shows that the eggs and mineral water combination are the most frequently occurring items when the length of the itemset is two.
Similarly, we can find the most frequently occurring items when the itemset length is 3:
# printing the frequntly items with length 3 frequent_itemsets[ (frequent_itemsets[‘length’] == 3) ].head(3)
Output:
The output shows that the most frequent items with a length of three are eggs, spaghetti, and mineral water.
Mining association rules
We know that the association rules are simply the if-else statements. The IF component of an association rule is known as the antecedent. The THEN component is known as the consequent. The antecedent and the consequent are disjoint; they have no items in common.
So, let’s create antecedents and consequents:
# We set our metric as “Lift” to define whether antecedents & consequents are dependent our not rules = association_rules(frequent_itemsets, metric=”lift”, min_threshold=1.2) rules[“antecedents_length”] = rules[“antecedents”].apply(lambda x: len(x)) rules[“consequents_length”] = rules[“consequents”].apply(lambda x: len(x)) rules.sort_values(“lift”,ascending=False)
Output:
The output above shows the values of various supporting components. To get more insights from the data, let’s sort the data by the confidence value:
# Sort values based on confidence rules.sort_values(“confidence”,ascending=False)
Output:
This table shows the relationship between different items and the likelihood of a customer buying those items together. For example, according to the table above, the customers who purchased eggs and ground beef are expected to buy mineral water with a likelihood of 50% (confidence).
Summary
The Apriori algorithm is a powerful tool for finding relationships between items in a dataset. In this article, we have covered the basics of the algorithm and shown how to implement it in Python. We hope you find this information useful and that you are now able to use the Apriori algorithm to discover valuable insights from your own data sets.
Related articles
So you have finished reading the apriori 알고리즘 파이썬 topic article, if you find this article useful, please share it. Thank you very much. See more: Apriori 알고리즘 구현, 파이썬 데이터 분석 알고리즘, 아프리오리 알고리즘, Apriori 알고리즘, Apriori leverage conviction, Association 분석, 장바구니 분석 SQL, A priori 파이썬