You are looking for information, articles, knowledge about the topic nail salons open on sunday near me qnorm pnorm on Google, you do not find the information you need! Here are the best content compiled and compiled by the https://chewathai27.com/to team, along with other related topics such as: qnorm pnorm Pnorm, R pnorm, Qnorm, Pnorm in R, Qnorm in R, Rnorm in R, Dnorm in R, Punif in R
Using probability distributions in R: dnorm, pnorm, qnorm, and rnorm – Data Science Blog: Understand. Implement. Succed.
- Article author: www.datascienceblog.net
- Reviews from users: 42843
Ratings
- Top rated: 4.4
- Lowest rated: 1
- Summary of article content: Articles about Using probability distributions in R: dnorm, pnorm, qnorm, and rnorm – Data Science Blog: Understand. Implement. Succed. Of the four functions dealing with distributions, dnorm is the most important one. This is because the values from pnorm , qnorm , and rnorm are … …
- Most searched keywords: Whether you are looking for Using probability distributions in R: dnorm, pnorm, qnorm, and rnorm – Data Science Blog: Understand. Implement. Succed. Of the four functions dealing with distributions, dnorm is the most important one. This is because the values from pnorm , qnorm , and rnorm are … R is made for working with distributions. Learn how to work with the density function and more here!
- Table of Contents:
Distribution functions in R
The probability density function dnorm
The cumulative density function pnorm
The quantile function qnorm
The random sampling function rnorm
Summary
Comments

Introduction to dnorm, pnorm, qnorm, and rnorm for new biostatisticians
- Article author: seankross.com
- Reviews from users: 40421
Ratings
- Top rated: 4.0
- Lowest rated: 1
- Summary of article content: Articles about Introduction to dnorm, pnorm, qnorm, and rnorm for new biostatisticians The function pnorm returns the integral from −∞ to q of the pdf of the normal distribution where q is a Z-score. Try to guess the value of … …
- Most searched keywords: Whether you are looking for Introduction to dnorm, pnorm, qnorm, and rnorm for new biostatisticians The function pnorm returns the integral from −∞ to q of the pdf of the normal distribution where q is a Z-score. Try to guess the value of …
- Table of Contents:
dnorm
pnorm
qnorm
rnorm
Closing thoughts

NORMAL DISTRIBUTION in R 🔔 [dnorm, pnorm, qnorm and rnorm]
- Article author: r-coder.com
- Reviews from users: 46294
Ratings
- Top rated: 3.1
- Lowest rated: 1
- Summary of article content: Articles about NORMAL DISTRIBUTION in R 🔔 [dnorm, pnorm, qnorm and rnorm] The pnorm function gives the Cumulative Distribution Function (CDF) of the Normal distribution in R, which is the probability that the variable X X X takes a … …
- Most searched keywords: Whether you are looking for NORMAL DISTRIBUTION in R 🔔 [dnorm, pnorm, qnorm and rnorm] The pnorm function gives the Cumulative Distribution Function (CDF) of the Normal distribution in R, which is the probability that the variable X X X takes a … Normal Distribution in R. Plot a normal distribution, calculate dnorm, pnorm, qnorm and rnorm functions and know the differences between them
- Table of Contents:
The normal or gaussian distribution
The dnorm function
The pnorm function
The qnorm function
The rnorm function
![NORMAL DISTRIBUTION in R 🔔 [dnorm, pnorm, qnorm and rnorm]](https://r-coder.com/wp-content/uploads/2020/06/normal-distribution-r.png)
A Guide to dnorm, pnorm, qnorm, and rnorm in R – Statology
- Article author: www.statology.org
- Reviews from users: 17515
Ratings
- Top rated: 3.9
- Lowest rated: 1
- Summary of article content: Articles about A Guide to dnorm, pnorm, qnorm, and rnorm in R – Statology This tutorial explains how to work with the normal distribution in R using the functions dnorm, pnorm, rnorm, and qnorm. …
- Most searched keywords: Whether you are looking for A Guide to dnorm, pnorm, qnorm, and rnorm in R – Statology This tutorial explains how to work with the normal distribution in R using the functions dnorm, pnorm, rnorm, and qnorm. A simple guide to the functions dnorm, pnorm, rnorm, and qnorm in R.
- Table of Contents:
dnorm
pnorm
qnorm
rnorm
Published by Zach
Post navigation
Search
ABOUT
Statology Study
Introduction to Statistics Course
Recent Posts

Probability distributions
- Article author: thomasleeper.com
- Reviews from users: 12750
Ratings
- Top rated: 4.7
- Lowest rated: 1
- Summary of article content: Articles about Probability distributions For example, the dnorm function proves the density of the normal distribution at a specific quantile. The pnorm function proves the cumulative density of … …
- Most searched keywords: Whether you are looking for Probability distributions For example, the dnorm function proves the density of the normal distribution at a specific quantile. The pnorm function proves the cumulative density of …
- Table of Contents:
Density functions
Cumulative distribution functions
Quantile function
Other distributions

A Guide to dnorm, pnorm, rnorm, and qnorm in R – GeeksforGeeks
- Article author: www.geeksforgeeks.org
- Reviews from users: 11170
Ratings
- Top rated: 4.6
- Lowest rated: 1
- Summary of article content: Articles about A Guide to dnorm, pnorm, rnorm, and qnorm in R – GeeksforGeeks pnorm function. This function returns the value of the cumulative density function (cdf) of the normal distribution given a certain random … …
- Most searched keywords: Whether you are looking for A Guide to dnorm, pnorm, rnorm, and qnorm in R – GeeksforGeeks pnorm function. This function returns the value of the cumulative density function (cdf) of the normal distribution given a certain random … Data Structures,Algorithms,Python,C,C++,Java,JavaScript,How to,Android Development,SQL,C#,PHP,Golang,Data Science,Machine Learning,PHP,Web Development,System Design,Tutorial,Technical Blogs,School Learning,Interview Experience,Interview Preparation,Programming,Competitive Programming,SDE Sheet,Jobathon,Coding Contests,GATE CSE,Placement,Learn To Code,Aptitude,Quiz,Tips,CSS,HTML,jQuery,Bootstrap,MySQL,NodeJS,React,Angular,Tutorials,Courses,Learn to code,Source codeA Computer Science portal for geeks. It contains well written, well thought and well explained computer science and programming articles, quizzes and practice/competitive programming/company interview Questions.
- Table of Contents:
Related Articles
dnorm function
R
pnorm function
R
qnorm function
R
rnorm function
R
Start Your Coding Journey Now!
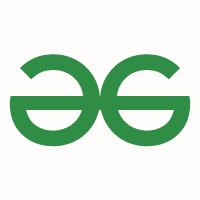
Normal Distribution in R (Example) | dnorm, pnorm, qnorm, rnorm Function
- Article author: statisticsglobe.com
- Reviews from users: 39775
Ratings
- Top rated: 3.0
- Lowest rated: 1
- Summary of article content: Articles about Normal Distribution in R (Example) | dnorm, pnorm, qnorm, rnorm Function How to apply the normal distribution functions in R – 5 programming examples – dnorm, pnorm, qnorm & rnorm functions explained – Plot & random numbers. …
- Most searched keywords: Whether you are looking for Normal Distribution in R (Example) | dnorm, pnorm, qnorm, rnorm Function How to apply the normal distribution functions in R – 5 programming examples – dnorm, pnorm, qnorm & rnorm functions explained – Plot & random numbers. How to apply the normal distribution functions in R – 5 programming examples – dnorm, pnorm, qnorm & rnorm functions explained – Plot & random numbers
- Table of Contents:
Example 1 Normally Distributed Density (dnorm Function)
Example 2 Distribution Function (pnorm Function)
Example 3 Quantile Function (qnorm Function)
Example 4 Random Number Generation (rnorm Function)
Example 5 Modify Mean & Standard Deviation
Video Further Resources & Summary

Normal function – RDocumentation
- Article author: www.rdocumentation.org
- Reviews from users: 39935
Ratings
- Top rated: 3.1
- Lowest rated: 1
- Summary of article content: Articles about Normal function – RDocumentation dnorm gives the density, pnorm gives the distribution function, qnorm gives the quantile function, and rnorm generates random deviates. …
- Most searched keywords: Whether you are looking for Normal function – RDocumentation dnorm gives the density, pnorm gives the distribution function, qnorm gives the quantile function, and rnorm generates random deviates.
Density, distribution function, quantile function and random
generation for the normal distribution with mean equal tomean
and standard deviation equal tosd
. - Table of Contents:
Description
Usage
Arguments
Value
Details
References
See Also
Examples

qnorm pnorm
- Article author: timoday.edu.vn
- Reviews from users: 35690
Ratings
- Top rated: 3.9
- Lowest rated: 1
- Summary of article content: Articles about qnorm pnorm pnorm(q, mean, sd) qnorm(p, mean, sd) rnorm(n, mean, sd). Nhị phân dbinom(k, n, p) pbinom(q, n, p) qbinom (p, n, p) rbinom(k, n, prob). Poisson. …
- Most searched keywords: Whether you are looking for qnorm pnorm pnorm(q, mean, sd) qnorm(p, mean, sd) rnorm(n, mean, sd). Nhị phân dbinom(k, n, p) pbinom(q, n, p) qbinom (p, n, p) rbinom(k, n, prob). Poisson.
- Table of Contents:

See more articles in the same category here: Chewathai27.com/to/blog.
Using probability distributions in R: dnorm, pnorm, qnorm, and rnorm
Distribution functions in R Every distribution has four associated functions whose prefix indicates the type of function and the suffix indicates the distribution. To exemplify the use of these functions, I will limit myself to the normal (Gaussian) distribution. The four normal distribution functions are: d norm: density function of the normal distribution
norm: of the normal distribution p norm: cumulative density function of the normal distribution
norm: of the normal distribution q norm: quantile function of the normal distribution
norm: of the normal distribution rnorm: random sampling from the normal distribution
The probability density function: dnorm The probability density function (PDF, in short: density) indicates the probability of observing a measurement with a specific value and thus the integral over the density is always 1. For a value \(x\), the normal density is defined as \[{\displaystyle f(x\mid \mu ,\sigma ^{2})={\frac {1}{\sqrt {2\pi \sigma ^{2}}}}\text{exp}\left(-{\frac {(x-\mu )^{2}}{2\sigma ^{2}}}\right)}\] where \(\mu\) is the mean, \(\sigma\) is the standard deviation, and \(\sigma^2\) is the variance. Using the density, it is possible to determine the probabilities of events. For example, you may wonder: What is the likelihood that a person has an IQ of exactly 140?. In this case, you would need to retrieve the density of the IQ distribution at value 140. The IQ distribution can be modeled with a mean of 100 and a standard deviation of 15. The corresponding density is: sample.range <- 50:150 iq.mean <- 100 iq.sd <- 15 iq.dist <- dnorm(sample.range, mean = iq.mean, sd = iq.sd) iq.df <- data.frame("IQ" = sample.range, "Density" = iq.dist) library(ggplot2) ggplot(iq.df, aes(x = IQ, y = Density)) + geom_point() From these data, we can now answer the initial question as well as additional questions: pp <- function(x) { print(paste0(round(x * 100, 3), "%")) } # likelihood of IQ == 140? pp(iq.df$Density[iq.df$IQ == 140]) ## [1] "0.076%" # likelihood of IQ >= 140? pp(sum(iq.df$Density[iq.df$IQ >= 140])) ## [1] “0.384%” # likelihood of 50 < IQ <= 90? pp(sum(iq.df$Density[iq.df$IQ <= 90])) ## [1] "26.284%" The cumulative density function: pnorm The cumulative density (CDF) function is a monotonically increasing function as it integrates over densities via \[f(x | \mu, \sigma) = \displaystyle {\frac {1}{2}}\left[1+\operatorname {erf} \left({\frac {x-\mu }{\sigma {\sqrt {2}}}}\right)\right]\] where \(\text{erf}(x) = \frac {1}{\sqrt {\pi }}\int _{-x}^{x}e^{-t^{2}}\,dt\) is the error function. To get an intuition of the CDF, let’s create a plot for the IQ data: cdf <- pnorm(sample.range, iq.mean, iq.sd) iq.df <- cbind(iq.df, "CDF_LowerTail" = cdf) ggplot(iq.df, aes(x = IQ, y = CDF_LowerTail)) + geom_point() As we can see, the depicted CDF shows the probability of having an IQ less or equal to a given value. This is because pnorm computes the lower tail by default, i.e. \(P[X <= x]\). Using this knowledge, we can obtain answers to some of our previous questions in a slightly different manner: # likelihood of 50 < IQ <= 90? pp(iq.df$CDF_LowerTail[iq.df$IQ == 90]) ## [1] "25.249%" # set lower.tail to FALSE to obtain P[X >= x] cdf <- pnorm(sample.range, iq.mean, iq.sd, lower.tail = FALSE) iq.df <- cbind(iq.df, "CDF_UpperTail" = cdf) # Probability for IQ >= 140? same value as before using dnorm! pp(iq.df$CDF_UpperTail[iq.df$IQ == 140]) ## [1] “0.383%” Note that the results from pnorm are the same as those obtained from manually summing up the probabilities obtained via dnorm. Moreover, by setting lower.tail = FALSE , dnorm can be used to directly compute p-values, which measure how the likelihood of an observation that is at least as extreme as the obtained one. To remember that pnorm does not provide the PDF but the CDF, just imagine that the function carries a p in its name such that pnorm is lexicographically close to qnorm, which provides the inverse of the CDF.
The quantile function: qnorm The quantile function is simply the inverse of the cumulative density function (iCDF). Thus, the quantile function maps from probabilities to values. Let’s take a look at the quantile function for \(P[X <= x]\): # input to qnorm is a vector of probabilities prob.range <- seq(0, 1, 0.001) icdf.df <- data.frame("Probability" = prob.range, "IQ" = qnorm(prob.range, iq.mean, iq.sd)) ggplot(icdf.df, aes(x = Probability, y = IQ)) + geom_point() Using the quantile function, we can answer quantile-related questions: # what is the 25th IQ percentile? print(icdf.df$IQ[icdf.df$Probability == 0.25]) ## [1] 89.88265 # what is the 75 IQ percentile? print(icdf.df$IQ[icdf.df$Probability == 0.75]) ## [1] 110.1173 # note: this is the same results as from the quantile function quantile(icdf.df$IQ) ## 0% 25% 50% 75% 100% ## -Inf 89.88265 100.00000 110.11735 Inf The random sampling function: rnorm When you want to draw random samples from the normal distribution, you can use rnorm . For example, we could use rnorm to simulate random samples from the IQ distribution. # fix random seed for reproducibility set.seed(1) # law of large numbers: mean will approach expected value for large N n.samples <- c(100, 1000, 10000) my.df <- do.call(rbind, lapply(n.samples, function(x) data.frame("SampleSize" = x, "IQ" = rnorm(x, iq.mean, iq.sd)))) # show one facet per random sample of a given size ggplot() + geom_histogram(data = my.df, aes(x = IQ)) + facet_wrap(.~SampleSize, scales = "free_y") # note: we can also implement our own sampler using the densities my.sample <- sample(iq.df$IQ, 100, prob = iq.df$Density, replace = TRUE) my.sample.df <- data.frame("IQ" = my.sample) ggplot(my.sample.df, aes(x = IQ)) + geom_histogram() Note that we called set.seed in order to ensure that the random number generator always generates the same sequence of numbers for reproducibility.
NORMAL DISTRIBUTION in R 🔔 [dnorm, pnorm, qnorm and rnorm]
The Normal or Gaussian distribution is the most known and important distribution in Statistics. In this tutorial you will learn what are and what does dnorm, pnorm, qnorm and rnorm functions in R and the differences between them. In consequence, you will learn how to create and plot the Normal distribution in R, calculate probabilities under the curves, the quantiles, Normal random sampling and even how to shade a specific area under a Normal curve.
The normal or gaussian distribution
Among continuous random variables, the most important is the Normal or Gaussian distribution. This variable was introduced by Carl Friedrich in the XIX century for studying error measures.
Let X \sim N(\mu, \sigma), namely a random variable following a normal distribution with mean \mu and standard deviation \sigma:
The probability density function (PDF), also known as Bell curve, of x is f(x) = \frac{1}{\sqrt{2\pi \sigma^{2}}} e^{\frac{1}{2} (\frac{x – \mu}{\sigma})^2} .
(PDF), also known as Bell curve, of is . The cumulative distribution function (CDF) is F(x) = P(X \leq x) .
(CDF) is . The quantile function is Q(p) = F^{-1}(p) .
is . The expencted mean and variance are E(X) = \mu and Var(X) = \sigma^2 , respectively.
In R there exist the dnorm , pnorm and qnorm functions, which allows calculating the normal density, distribution and quantile function for a set of values. In addition, the rnorm function allows obtaining random observations that follow a normal distibution. The following table summarizes the functions related to the normal distribution:
Function Description dnorm Normal density
(Probability Density Function) pnorm Normal distribution
(Cumulative Distribution Function) qnorm Quantile function of the Normal distribution rnorm Normal random number generation
By default, these functions consider the standard Normal distribution, which has a mean of zero and a standard deviation of one.
Although we will review each function in detail on the corresponding section, in the following illustration you can appreciate the relationship between the dnorm , pnorm and qnorm functions.
The dnorm function
In R, you can make use of the dnorm function to calculate the density function with mean \mu and standard deviation \sigma for any value of x, \mu and \sigma.
dnorm(x, # X-axis values (grid) mean = 0, # Integer or vector representing the mean/s sd = 1, # Integer or vector representing the standard deviation/s log = FALSE) # If TRUE, probabilities are given as log
Consider, for instance, that you want to obtain the PDF for x \in (-4, 4), with mean 1 and standard deviation of 3. In order to calculate it, you could type:
x <- -4:4 # x <- seq(-4, 4, length = 100) # More data points dnorm(x, mean = 1, sd = 3) 0.03315905 0.05467002 0.08065691 0.10648267 0.12579441 0.13298076 0.12579441 0.10648267 0.08065691 You can also specify vectors to the mean and sd arguments of the function. In the following example, the value of even elements are from \mu = 1, \sigma = 3 and odds are from \mu = 2, \sigma = 4. dnorm(x, mean = c(1, 2), sd = c(3, 4)) 0.03315905 0.04566227 0.08065691 0.07528436 0.12579441 0.09666703 0.12579441 0.09666703 0.08065691 Plot Normal distribution in R Creating a normal distribution plot in R is easy. You just need to create a grid for the X-axis for the first argument of the plot function and pass as input of the second the dnorm function for the corresponding grid. In the following example we show how to plot normal distributions for different means and variances. par(mfrow = c(1, 2)) # Grid of X-axis values x <- seq(-4, 8, 0.1) #----------------------------------------- # Same standard deviation, different mean #----------------------------------------- # Mean 0, sd 1 plot(x, dnorm(x, mean = 0, sd = 1), type = "l", ylim = c(0, 0.6), ylab = "", lwd = 2, col = "red") # Mean 3, sd 1 lines(x, dnorm(x, mean = 3, sd = 1), col = "blue", lty = 1, lwd = 2) # Adding a legend legend("topright", legend = c("0 1", "3 1"), col = c("red", "blue"), title = expression(paste(mu, " ", sigma)), title.adj = 0.9, lty = 1, lwd = 2, box.lty = 0) #----------------------------------------- # Same mean, different standard deviation #----------------------------------------- # Mean 1, sd 1 plot(x, dnorm(x, mean = 1, sd = 1), type = "l", ylim = c(0, 1), ylab = "", lwd = 2, col = "red") # Mean 1, sd 0.5 lines(x, dnorm(x, mean = 1, sd = 0.5), col = "blue", lty = 1, lwd = 2) # Adding a legend legend("topright", legend = c("1 1", "1 0.5"), col = c("red", "blue"), title = expression(paste(mu, " ", sigma)), title.adj = 0.75, lty = 1, lwd = 2, box.lty = 0) par(mfrow = c(1, 1)) The pnorm function The pnorm function gives the Cumulative Distribution Function (CDF) of the Normal distribution in R, which is the probability that the variable X takes a value lower or equal to x. The syntax of the function is the following: pnorm(q, mean = 0, sd = 1, lower.tail = TRUE, # If TRUE, probabilities are P(X <= x), or P(X > x) otherwise log.p = FALSE) # If TRUE, probabilities are given as log
As an example, taking into account that the Normal distribution is symmetric, the probability that the variable will take a value lower than the mean is 0.5:
pnorm(0, mean = 0, sd = 1) # 0.5
pnorm function example
Now, suppose that you have a machine that packages rice inside boxes. The process follows a Normal distribution and it is known that the mean of the weight of each box is 1000 grams and the standard deviation is 10 grams. You can plot the density function typing:
Mean <- 1000 Sd <- 10 # X grid for non-standard normal distribution x <- seq(-3, 3, length = 100) * Sd + Mean # Density function f <- dnorm(x, Mean, Sd) plot(x, f, type = "l", lwd = 2, col = "blue", ylab = "", xlab = "Weight") abline(v = Mean) # Vertical line on the mean First, if you want to calculate the probability of a box weighing less than 1010 grams (P(X < 1010) = P(X \leq 1010)), you can type the following: pnorm(1010, Mean, Sd) # 0.8413447 or 84.13% 1 - pnorm(1010, Mean, Sd, lower.tail = FALSE) # Equivalent So the probability of a box wheighing less than 1010 grams is 0.8413 or 84.13%, which corresponds to the following area: lb <- min(x) # Lower bound ub <- 1010 # Upper bound x2 <- seq(min(x), ub, length = 100) # New Grid y <- dnorm(x2, Mean, Sd) # Density plot(x, f, type = "l", lwd = 2, col = "blue", ylab = "", xlab = "Weight") abline(v = ub) polygon(c(lb, x2, ub), c(0, y, 0), col = rgb(0, 0, 1, alpha = 0.5)) text(995, 0.01, "84.13%") As shading the area under the Normal curve can be tricky and requires several lines of code, we have created a simple function to achieve it in a single line: # mean: mean of the Normal variable # sd: standard deviation of the Normal variable # lb: lower bound of the area # ub: upper bound of the area # acolor: color of the area # ...: additional arguments to be passed to lines function normal_area <- function(mean = 0, sd = 1, lb, ub, acolor = "lightgray", ...) { x <- seq(mean - 3 * sd, mean + 3 * sd, length = 100) if (missing(lb)) { lb <- min(x) } if (missing(ub)) { ub <- max(x) } x2 <- seq(lb, ub, length = 100) plot(x, dnorm(x, mean, sd), type = "n", ylab = "") y <- dnorm(x2, mean, sd) polygon(c(lb, x2, ub), c(0, y, 0), col = acolor) lines(x, dnorm(x, mean, sd), type = "l", ...) } As an example, if you want to shade the area between -1 and 2 of a standard Normal distribution you can type: normal_area(mean = 0, sd = 1, lb = -1, ub = 2, lwd = 2) Second, in case that you want to calculate the probability of a box weighing more than 980 grams (P(X > 980) = P(X \geq 980)) you can use the lower.tail argument.
pnorm(980, Mean, Sd, lower.tail = FALSE) # 0.9772499 or 97.72% 1 – pnorm(980, Mean, Sd) # Equivalent pnorm(1020, Mean, Sd) # Equivalent by symmetry
The calculated probability corresponds to the following area:
normal_area(mean = Mean, sd = Sd, lb = 980, acolor = rgb(0, 0, 1, alpha = 0.5)) text(1000, 0.01, “97.72%”)
Finally, if you want to calculate the probability of a box weighing more than 990 grams and less than 1000 you have to calculate P(X \leq 1000) – P(x \leq 990) = P(X < 1000) - P(x <990) and hence you can type: pnorm(1000, Mean, Sd) - pnorm(990, Mean, Sd) # 0.3413447 or 34.13% You can plot the area with the following code: normal_area(mean = Mean, sd = Sd, lb = 990, ub = 1000, acolor = rgb(0, 0, 1, alpha = 0.5)) text(995, 0.01, "34.13%", srt = 90) For continuous variables, as P(X = x) = 0 , P(X \geq x) = P(X > x) and P(X \leq x) = P(X < x) . Plot normal cumulative distribution function in R With the pnorm function you can also plot the cumulative density function of the Gaussian or Normal distribution in R: par(mfrow = c(1, 2)) # Grid of X-axis values x <- seq(-4, 8, 0.1) #----------------------------------------- # Same standard deviation, different mean #----------------------------------------- # Mean 0, sd 1 plot(x, pnorm(x, mean = 0, sd = 1), type = "l", ylim = c(0, 1), ylab = "", lwd = 2, col = "red") # Mean 3, sd 1 lines(x, pnorm(x, mean = 3, sd = 1), col = "blue", lty = 1, lwd = 2) # Legend legend("topleft", legend = c("0 1", "3 1"), col = c("red", "blue"), title = expression(paste(mu, " ", sigma)), title.adj = 0.9, lty = 1, lwd = 2, box.lty = 0) #----------------------------------------- # Same mean, different standard deviation #----------------------------------------- # Mean 1, sd 1 plot(x, pnorm(x, mean = 1, sd = 1), type = "l", ylim = c(0, 1), ylab = "", lwd = 2, col = "red") # Mean 1, sd 0.5 lines(x, pnorm(x, mean = 1, sd = 0.5), col = "blue", lty = 1, lwd = 2) # Legend legend("topleft", legend = c("1 1", "1 0.5"), col = c("red", "blue"), title = expression(paste(mu, " ", sigma)), title.adj = 0.75, lty = 1, lwd = 2, box.lty = 0) par(mfrow = c(1, 1)) Recall that P(X < 0) = 0.5 for a standard Normal distribution: x <- seq(-4, 4, 0.1) plot(x, pnorm(x, mean = 0, sd = 1), type = "l", ylim = c(0, 1), ylab = "P(X < x)", lwd = 2, col = "red") segments(0, 0, 0, 0.5, lwd = 2, lty = 2) segments(-4, 0.5, 0, 0.5, lwd = 2, lty = 2) The qnorm function The qnorm function allows you to find the quantile (percentile) Q for any probability p. Hence, the qnorm function is the inverse of the pnorm function. The syntax of qnorm is as follows: qnorm(p, # Integer or vector of probabilities mean = 0, # Integer or vector of means sd = 1, # Integer or vector of standard deviations lower.tail = TRUE, # If TRUE, probabilities are P(X <= x), or P(X > x) otherwise log.p = FALSE) # If TRUE, probabilities are given as log
As a first example, the quantile for probability 0.5 (Q(0.5)) on a symmetric distribution is equal to the mean:
qnorm(0.5, mean = 0, sd = 1) # 0
In addition, you can obtain the quantile for any given probability. Note the relation between pnorm and qnorm functions:
x <- pnorm(-1.5, mean = 0, sd = 1) # 0.0668072 qnorm(x, mean = 0, sd = 1) # -1.5 normal_area(mean = 0, sd = 1, ub = -1.5, lwd = 2, acolor = rgb(0, 0, 1, alpha = 0.5)) arrows(-0.5, 0.1, -1.45, 0, lwd = 2, length = 0.2) text(-0.25, 0.13, "-1.5", cex = 1.5) If you want to calculate, for instance, the quantile Q(P(X > 1.5)) = Q(1 – P(X \leq 1.5)) = Q(0.067) you can set the lower.tail argument to TRUE .
x <- pnorm(1.5, mean = 0, sd = 1, lower.tail = FALSE) # 0.0668072 qnorm(x, mean = 0, sd = 1, lower.tail = FALSE) # 1.5 # Equivalent to: # x <- 1- pnorm(1.5, mean = 0, sd = 1) # qnorm(1 - x, mean = 0, sd = 1) normal_area(mean = 0, sd = 1, lb = 1.5, lwd = 2, acolor = rgb(0, 0, 1, alpha = 0.5)) arrows(0, 0.1, 1.45, 0, lwd = 2, length = 0.2) text(0, 0.13, "1.5", cex = 1.5) Plotting the Normal quantile function You can plot the quantile function of a standard Normal distribution typing the following: plot(qnorm, pnorm(-4), pnorm(4), lwd = 2, xlab = "p", ylab = "Q(p)") # Equivalent to: # x <- seq(pnorm(-4), pnorm(4), length = 100) # plot(x, qnorm(x, mean = 0, sd = 1), type = "l", # lwd = 2, xlab = "p", ylab = "Q(p)") The previous plot represents the possible outcomes of the qnorm function for the standard Normal distribution. Recall that Q(0.5) = 0: plot(qnorm, pnorm(-4), pnorm(4), lwd = 2, xlab = "p", ylab = "Q(p)") segments(0.5, -4, 0.5, 0, lty = 2, lwd = 2) segments(0, 0, 0.5, 0, lty = 2, lwd = 2) The rnorm function The rnorm function generates n observations from the Normal distribution with mean \mu and standard deviation \sigma. The syntax of the rnorm function in R is the following: rnorm(n, # Number of observations to be generated mean = 0, # Integer or vector of means sd = 1) # Integer or vector of standard deviations Hence, you can generate 10 observations of a standard Normal distribution in R with the following code: rnorm(10) 0.3320065 -0.6454991 -0.3692699 -0.4160260 0.5756340 -0.8793241 -0.8095590 0.4926698 -0.8038873 -0.3446890 However, it should be noted that if you don’t specify a seed the output won’t be reproducible. You can make use of the set.seed function to make your code reproducible: # Seed for reproducibility set.seed(1) rnorm(1) # -0.6264538 In addition, in the following plot you can observe how increasing the number of observations, the histogram of the data approaches to the true Normal density function:
A Guide to dnorm, pnorm, qnorm, and rnorm in R
The normal distribution is the most commonly used distribution in statistics. This tutorial explains how to work with the normal distribution in R using the functions dnorm, pnorm, rnorm, and qnorm.
dnorm
The function dnorm returns the value of the probability density function (pdf) of the normal distribution given a certain random variable x, a population mean μ and population standard deviation σ. The syntax for using dnorm is as follows:
dnorm(x, mean, sd)
The following code illustrates a few examples of dnorm in action:
#find the value of the standard normal distribution pdf at x=0 dnorm(x=0, mean=0, sd=1) # [1] 0.3989423 #by default, R uses mean=0 and sd=1 dnorm(x=0) # [1] 0.3989423 #find the value of the normal distribution pdf at x=10 with mean=20 and sd=5 dnorm(x=10, mean=20, sd=5) # [1] 0.01079819
Typically when you’re trying to solve questions about probability using the normal distribution, you’ll often use pnorm instead of dnorm. One useful application of dnorm, however, is in creating a normal distribution plot in R. The following code illustrates how to do so:
#Create a sequence of 100 equally spaced numbers between -4 and 4 x <- seq(-4, 4, length=100) #create a vector of values that shows the height of the probability distribution #for each value in x y <- dnorm(x) #plot x and y as a scatterplot with connected lines (type = "l") and add #an x-axis with custom labels plot(x,y, type = "l", lwd = 2, axes = FALSE, xlab = "", ylab = "") axis(1, at = -3:3, labels = c("-3s", "-2s", "-1s", "mean", "1s", "2s", "3s")) This generates the following plot: pnorm The function pnorm returns the value of the cumulative density function (cdf) of the normal distribution given a certain random variable q, a population mean μ and population standard deviation σ. The syntax for using pnorm is as follows: pnorm(q, mean, sd) Put simply, pnorm returns the area to the left of a given value x in the normal distribution. If you’re interested in the area to the right of a given value q, you can simply add the argument lower.tail = FALSE pnorm(q, mean, sd, lower.tail = FALSE) The following examples illustrates how to solve some probability questions using pnorm. Example 1: Suppose the height of males at a certain school is normally distributed with a mean of μ=70 inches and a standard deviation of σ = 2 inches. Approximately what percentage of males at this school are taller than 74 inches? #find percentage of males that are taller than 74 inches in a population with #mean = 70 and sd = 2 pnorm(74, mean=70, sd=2, lower.tail=FALSE) # [1] 0.02275013 At this school, 2.275% of males are taller than 74 inches. Example 2: Suppose the weight of a certain species of otters is normally distributed with a mean of μ=30 lbs and a standard deviation of σ = 5 lbs. Approximately what percentage of this species of otters weight less than 22 lbs? #find percentage of otters that weight less than 22 lbs in a population with #mean = 30 and sd = 5 pnorm(22, mean=30, sd=5) # [1] 0.05479929 Approximately 5.4799% of this species of otters weigh less than 22 lbs. Example 3: Suppose the height of plants in a certain region is normally distributed with a mean of μ=13 inches and a standard deviation of σ = 2 inches. Approximately what percentage of plants in this region are between 10 and 14 inches tall? #find percentage of plants that are less than 14 inches tall, then subtract the #percentage of plants that are less than 10 inches tall, based on a population #with mean = 13 and sd = 2 pnorm(14, mean=13, sd=2) - pnorm(10, mean=13, sd=2) # [1] 0.6246553 Approximately 62.4655% of plants in this region are between 10 and 14 inches tall. qnorm The function qnorm returns the value of the inverse cumulative density function (cdf) of the normal distribution given a certain random variable p, a population mean μ and population standard deviation σ. The syntax for using qnorm is as follows: qnorm(p, mean, sd) Put simply, you can use qnorm to find out what the Z-score is of the pth quantile of the normal distribution. The following code illustrates a few examples of qnorm in action: #find the Z-score of the 99th quantile of the standard normal distribution qnorm(.99, mean=0, sd=1) # [1] 2.326348 #by default, R uses mean=0 and sd=1 qnorm(.99) # [1] 2.326348 #find the Z-score of the 95th quantile of the standard normal distribution qnorm(.95) # [1] 1.644854 #find the Z-score of the 10th quantile of the standard normal distribution qnorm(.10) # [1] -1.281552 rnorm The function rnorm generates a vector of normally distributed random variables given a vector length n, a population mean μ and population standard deviation σ. The syntax for using rnorm is as follows: rnorm(n, mean, sd) The following code illustrates a few examples of rnorm in action: #generate a vector of 5 normally distributed random variables with mean=10 and sd=2 five <- rnorm(5, mean = 10, sd = 2) five # [1] 10.658117 8.613495 10.561760 11.123492 10.802768 #generate a vector of 1000 normally distributed random variables with mean=50 and sd=5 narrowDistribution <- rnorm(1000, mean = 50, sd = 15) #generate a vector of 1000 normally distributed random variables with mean=50 and sd=25 wideDistribution <- rnorm(1000, mean = 50, sd = 25) #generate two histograms to view these two distributions side by side, specify #50 bars in histogram and x-axis limits of -50 to 150 par(mfrow=c(1, 2)) #one row, two columns hist(narrowDistribution, breaks=50, xlim=c(-50, 150)) hist(wideDistribution, breaks=50, xlim=c(-50, 150)) This generates the following histograms: Notice how the wide distribution is much more spread out compared to the narrow distribution. This is because we specified the standard deviation in the wide distribution to be 25 compared to just 15 in the narrow distribution. Also notice that both histograms are centered around the mean of 50.
So you have finished reading the qnorm pnorm topic article, if you find this article useful, please share it. Thank you very much. See more: Pnorm, R pnorm, Qnorm, Pnorm in R, Qnorm in R, Rnorm in R, Dnorm in R, Punif in R