You are looking for information, articles, knowledge about the topic nail salons open on sunday near me 랜덤 포레스트 예제 on Google, you do not find the information you need! Here are the best content compiled and compiled by the Chewathai27.com/to team, along with other related topics such as: 랜덤 포레스트 예제 파이썬 랜덤포레스트 예제, 랜덤포레스트 활용, 랜덤포레스트 예측 파이썬, 랜덤포레스트 변수중요도, 랜덤포레스트 파이썬, 랜덤포레스트 class_weight, 랜덤포레스트 실습, 랜덤포레스트 회귀
랜덤 포레스트 원리와 구현 사이킷런 예제로 코드 실습해보기
- Article author: for-my-wealthy-life.tistory.com
- Reviews from users: 45911
Ratings
- Top rated: 3.3
- Lowest rated: 1
- Summary of article content: Articles about 랜덤 포레스트 원리와 구현 사이킷런 예제로 코드 실습해보기 예를 들어 feature가 30개가 있다면 그 중에 랜덤하게 5개만 뽑아서 트리를 하나 만들고, 또 다시 랜덤하게 5개의 feature를 뽑아서 두번째 트리를 만들고 … …
- Most searched keywords: Whether you are looking for 랜덤 포레스트 원리와 구현 사이킷런 예제로 코드 실습해보기 예를 들어 feature가 30개가 있다면 그 중에 랜덤하게 5개만 뽑아서 트리를 하나 만들고, 또 다시 랜덤하게 5개의 feature를 뽑아서 두번째 트리를 만들고 … 오늘은 앙상블 기법 중 하나인 랜덤포레스트에 대해 공부해봤다. 랜덤포레스트를 배우려면 일단 결정트리(Decision Tree)가 뭔지부터 알아야 한다. 결정 트리에 대한 자세한 내용은 아래 포스팅을 참고! 2021.06.1..
- Table of Contents:
태그
‘파이썬머신러닝’ Related Articles
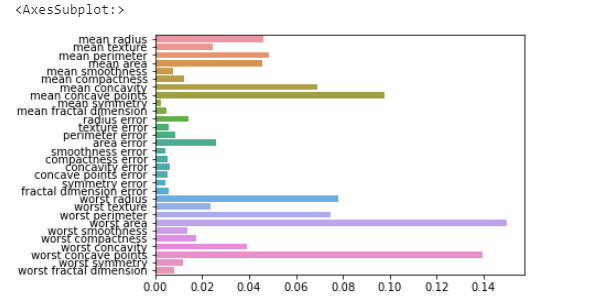
[머신러닝] 랜덤 포레스트 (Random Forest) 개념 및 예제 실습 / 독버섯 예제
- Article author: young2un.tistory.com
- Reviews from users: 2244
Ratings
- Top rated: 4.8
- Lowest rated: 1
- Summary of article content: Articles about [머신러닝] 랜덤 포레스트 (Random Forest) 개념 및 예제 실습 / 독버섯 예제 랜덤 포레스트 example · 한 줄이 버섯 한 종류 · 첫 번째 열 독 유무 p: poisionous, e: edible · 두 번째 열 버섯 머리 모양 b:벨, c:원뿔, x:볼록, f:평평 … …
- Most searched keywords: Whether you are looking for [머신러닝] 랜덤 포레스트 (Random Forest) 개념 및 예제 실습 / 독버섯 예제 랜덤 포레스트 example · 한 줄이 버섯 한 종류 · 첫 번째 열 독 유무 p: poisionous, e: edible · 두 번째 열 버섯 머리 모양 b:벨, c:원뿔, x:볼록, f:평평 … 랜덤 포레트스 랜덤 포레스트 – Random Forest 집단 학습을 기반으로 고정밀 분류, 회귀, 클러스트링 구현 학습 데이터로 다수의 의사결정 트리를 만들고 그 결과의 다수결 결과 유도로 높은 정밀도 무작위 샘플링..
- Table of Contents:
랜덤 포레스트 – Random Forest
태그
관련글
댓글0
공지사항
최근글
인기글
최근댓글
태그
전체 방문자
![[머신러닝] 랜덤 포레스트 (Random Forest) 개념 및 예제 실습 / 독버섯 예제](https://img1.daumcdn.net/thumb/R800x0/?scode=mtistory2&fname=https%3A%2F%2Fblog.kakaocdn.net%2Fdn%2FwyYaN%2FbtqFZ5z1gJx%2FNQ9XvanYOjk2rdkdUjJLE0%2Fimg.png)
[Python] Ensemble(앙상블) – Random Forest(랜덤포레스트)
- Article author: todayisbetterthanyesterday.tistory.com
- Reviews from users: 46275
Ratings
- Top rated: 4.3
- Lowest rated: 1
- Summary of article content: Articles about [Python] Ensemble(앙상블) – Random Forest(랜덤포레스트) 무작정 학습의 반복횟수/sample개수를 늘린다는 것이 성능의 향상을 장담하지는 않는다. 그렇기에 해당 데이터에 맞는 선정 모델의 parameter은 분석가가 … …
- Most searched keywords: Whether you are looking for [Python] Ensemble(앙상블) – Random Forest(랜덤포레스트) 무작정 학습의 반복횟수/sample개수를 늘린다는 것이 성능의 향상을 장담하지는 않는다. 그렇기에 해당 데이터에 맞는 선정 모델의 parameter은 분석가가 … 이 게시글은 오로지 파이썬을 통한 실습만을 진행한다. 앙상블 기법중 RandomForest의 개념 및 원리를 알고자하면 아래 링크를 통해학습을 진행하면 된다. https://todayisbetterthanyesterday.tistory.com/48?c..ML/DL 을 공부하고, ADP를 취득하기 위한 학부생의 공부노트입니다.
- Table of Contents:
![[Python] Ensemble(앙상블) - Random Forest(랜덤포레스트)](https://img1.daumcdn.net/thumb/R800x0/?scode=mtistory2&fname=https%3A%2F%2Fblog.kakaocdn.net%2Fdn%2FcLOGbz%2FbtqGc9o7ee9%2FETq2TCpW0FebE3hvKdZNp1%2Fimg.png)
[Machine Learning] Random Forest – 랜덤 포레스트 코드 구현 (feat. python)
- Article author: alex-blog.tistory.com
- Reviews from users: 46806
Ratings
- Top rated: 4.5
- Lowest rated: 1
- Summary of article content: Articles about [Machine Learning] Random Forest – 랜덤 포레스트 코드 구현 (feat. python) count, mean, std, min, 25%, 50%, 75%, max. age, 32561.0, 38.581647, 13.640433, 17.0, 28.0, 37.0, 48.0, 90.0. …
- Most searched keywords: Whether you are looking for [Machine Learning] Random Forest – 랜덤 포레스트 코드 구현 (feat. python) count, mean, std, min, 25%, 50%, 75%, max. age, 32561.0, 38.581647, 13.640433, 17.0, 28.0, 37.0, 48.0, 90.0. 1. 관련 라이브러리를 임포트. from IPython.core.display import display, HTML display(HTML(” “)) import numpy as np import pandas as pd import seaborn as sns from sklearn.metrics import classification..
- Table of Contents:
티스토리툴바
![[Machine Learning] Random Forest - 랜덤 포레스트 코드 구현 (feat. python)](https://t1.daumcdn.net/tistory_admin/static/images/openGraph/opengraph.png)
랜덤포레스트(Random Forest) :: BioinformaticsAndMe
- Article author: bioinformaticsandme.tistory.com
- Reviews from users: 15845
Ratings
- Top rated: 3.7
- Lowest rated: 1
- Summary of article content: Articles about 랜덤포레스트(Random Forest) :: BioinformaticsAndMe 1) Training set에서 표본 크기가 n인 bootstrap sampling 수행 · 2) Bootstrap sample에 대해 Random Forest Tree 모형 제작 · 3) Tree들의 앙상블 학습 … …
- Most searched keywords: Whether you are looking for 랜덤포레스트(Random Forest) :: BioinformaticsAndMe 1) Training set에서 표본 크기가 n인 bootstrap sampling 수행 · 2) Bootstrap sample에 대해 Random Forest Tree 모형 제작 · 3) Tree들의 앙상블 학습 … 랜덤포레스트(Random Forest) Start BioinformaticsAndMe 랜덤포레스트(Random Forest) : Random Forest는 오버피팅을 방지하기 위해, 최적의 기준 변수를 랜덤 선택하는 breiman(2001)이 제안한 머신러닝 기..Training is everything.
- Table of Contents:
랜덤포레스트(Random Forest)
티스토리툴바
[Python 분류] 랜덤포레스트(Random Forest) iris 데이터 예측 :: 마이자몽
- Article author: myjamong.tistory.com
- Reviews from users: 27543
Ratings
- Top rated: 3.6
- Lowest rated: 1
- Summary of article content: Articles about [Python 분류] 랜덤포레스트(Random Forest) iris 데이터 예측 :: 마이자몽 의사결정 트리(Decision Tree) 랜덤포레스트를 공부하기 전에 의사결정트리에 대해서 알아야한다. 말그대로 의사를 결정하는데 이진형 답변의 연속 … …
- Most searched keywords: Whether you are looking for [Python 분류] 랜덤포레스트(Random Forest) iris 데이터 예측 :: 마이자몽 의사결정 트리(Decision Tree) 랜덤포레스트를 공부하기 전에 의사결정트리에 대해서 알아야한다. 말그대로 의사를 결정하는데 이진형 답변의 연속 … 의사결정 트리(Decision Tree) 랜덤포레스트를 공부하기 전에 의사결정트리에 대해서 알아야한다. 말그대로 의사를 결정하는데 이진형 답변의 연속 모델이다. 쉬운 예시를 하나 들어보면 ‘스무고개’ 와 비슷하..
- Table of Contents:
태그
관련글
댓글0
최근글
인기글
티스토리툴바
랜덤포레스트 (Random Forest)
- Article author: data-workspace.tistory.com
- Reviews from users: 18814
Ratings
- Top rated: 4.2
- Lowest rated: 1
- Summary of article content: Articles about 랜덤포레스트 (Random Forest) 랜덤포레스트 ification 예제. 1) import pandas as pd import numpy as np from sklearn.ensemble import RandomForestClassifier from … …
- Most searched keywords: Whether you are looking for 랜덤포레스트 (Random Forest) 랜덤포레스트 ification 예제. 1) import pandas as pd import numpy as np from sklearn.ensemble import RandomForestClassifier from … 랜덤포레스트 (Random Forest) 1. 개념 – Decision Tree의 오버피팅 한계를 극복하기 위한 방법 – 데이터에 의사결정나무 여러 개를 동시에 적용해서 학습성능을 높이는 앙상블 기법 – 동일한 데이터로부터 복원..
- Table of Contents:
BASEMENT
랜덤포레스트 (Random Forest) 본문
랜덤포레스트 (Random Forest)
티스토리툴바
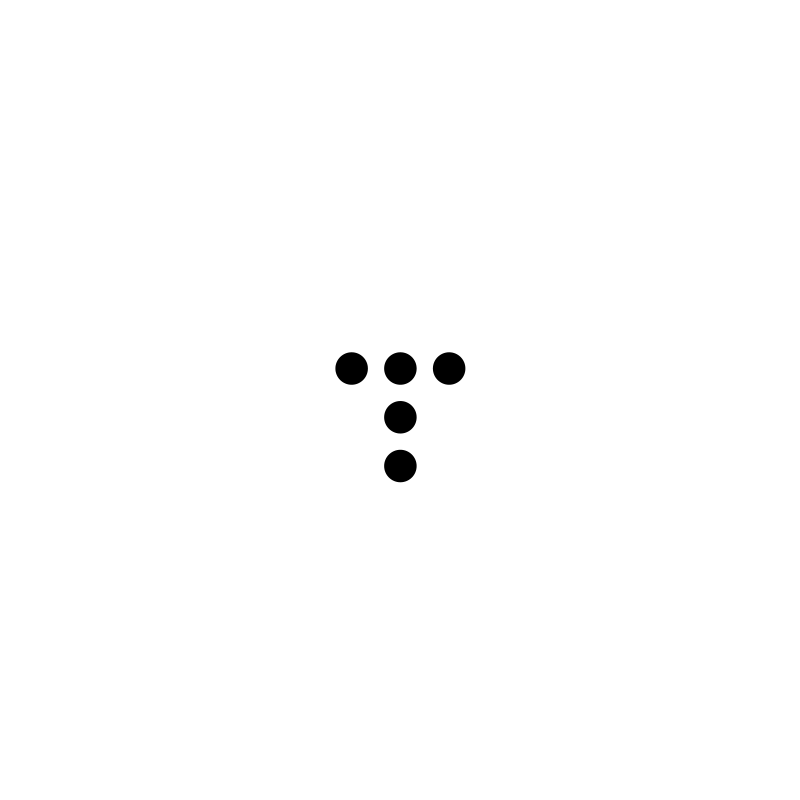
랜덤 포레스트(Random Forest)
- Article author: velog.io
- Reviews from users: 1107
Ratings
- Top rated: 4.6
- Lowest rated: 1
- Summary of article content: Articles about 랜덤 포레스트(Random Forest) 의사결정나무는 매우 훌륭한 모델이지만, 학습 데이터에 오버피팅하는 경향이 있다. 가지치기(pruning)같은 방법을 통해 그런 부작용을 최소화하는 전략이 … …
- Most searched keywords: Whether you are looking for 랜덤 포레스트(Random Forest) 의사결정나무는 매우 훌륭한 모델이지만, 학습 데이터에 오버피팅하는 경향이 있다. 가지치기(pruning)같은 방법을 통해 그런 부작용을 최소화하는 전략이 … 랜덤 포레스트
- Table of Contents:
머신러닝
배깅(Bagging)
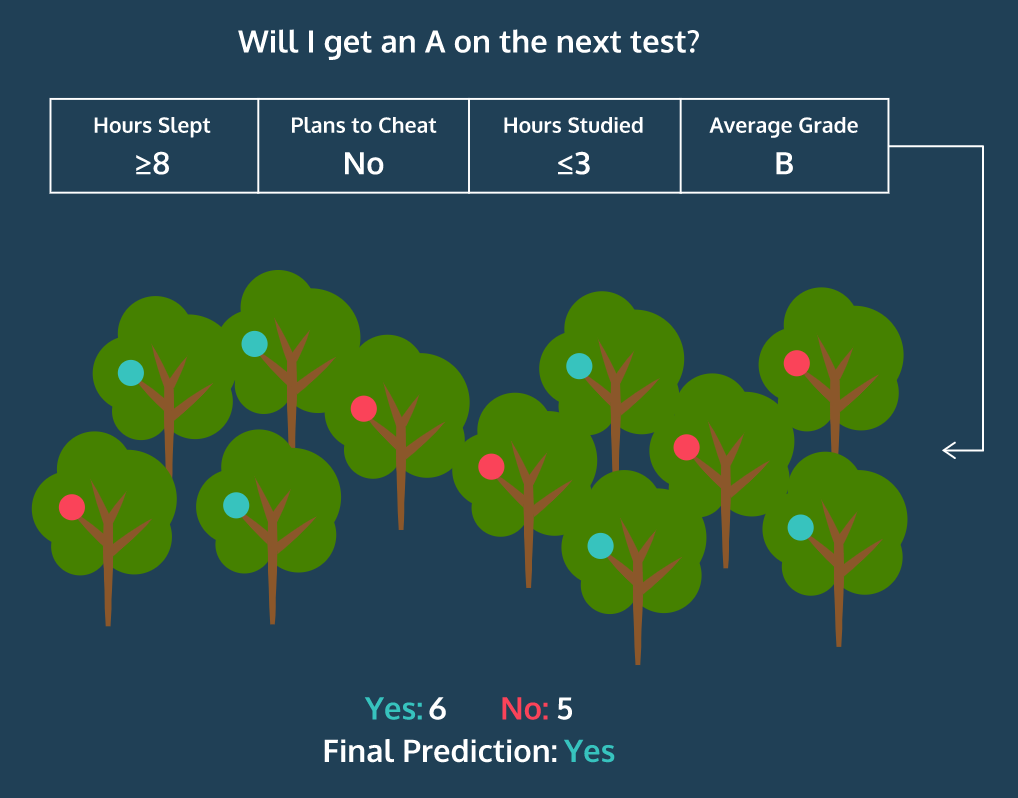
See more articles in the same category here: https://chewathai27.com/to/blog.
랜덤 포레스트 원리와 구현 사이킷런 예제로 코드 실습해보기
오늘은 앙상블 기법 중 하나인 랜덤포레스트에 대해 공부해봤다. 랜덤포레스트를 배우려면 일단 결정트리(Decision Tree)가 뭔지부터 알아야 한다. 결정 트리에 대한 자세한 내용은 아래 포스팅을 참고!
2021.06.13 – [파이썬/머신러닝] – 결정트리(Decision Tree) , 엔트로피 개념과 시각화까지
랜덤포레스트는 결정트리 여러개로 만들어진 모델이다. 결정트리는 훈련 데이터에 오버피팅된다는 치명적인 단점이 있기 때문에 보통은 결정트리 하나를 단독으로 사용하기 보다는 랜덤포레스트를 사용한다.
랜덤포레스트 개념
하나의 결정트리가 모든 feature를 변수로 사용해서 y값을 예측한다면 앞서 말한대로 오버피팅 문제가 발생한다. 그래서 랜덤포레스트는 feature를 무작위로 뽑거나, 데이터를 무작위로 뽑아서 여러개의 작은 트리를 만들고 그 트리들을 결합한다.
예를 들어 feature가 30개가 있다면 그 중에 랜덤하게 5개만 뽑아서 트리를 하나 만들고, 또 다시 랜덤하게 5개의 feature를 뽑아서 두번째 트리를 만들고, 이런 식으로 트리를 여러 개 만드는 것이 바로 랜덤포레스트이다.
트리를 여러 개 만들면 트리 개수만큼 예측 결과값이 생성되는데 voting을 통해 결과값을 채택하게 된다. 이게 바로 앙상블 학습이다.
랜덤포레스트는 기본적으로 결정트리의 단점을 보완하는 모델이라 오버피팅이 적고 성능이 뛰어나다. n_estimators, max_features, max_depth 정도와 같은 몇몇 파라미터만 잘 튜닝해주면 튜닝을 많이 하지 않아도 높은 성능을 보인다.
*앙상블: 의견을 통합하거나 여러 결과를 합치는 것
-분류모델: 가장 많이 나온 값
-회귀모델: 평균값
랜덤포레스트 주요 파라미터
n_estimators: 트리를 몇 개 만들 것인지 (int, default=100), 값이 클수록 오버피팅 방지
criterion: gini 또는 entropy 중 선택
max_depth: 트리의 깊이 (int, default=None)
bootstrap: True이면 전체 feature에서 복원추출해서 트리 생성 (default=True)
max_features: 선택할 feature의 개수, 보통 default값으로 씀 (default=’auto’)
출처: scikit-learn
랜덤포레스트 예제(실습 코드)
사이킷런의 breast_cancer 데이터로 간단하게 예제 코드를 실행해보자.
from sklearn.tree import DecisionTreeClassifier from sklearn.ensemble import RandomForestClassifier from sklearn.datasets import load_breast_cancer from sklearn.model_selection import train_test_split cancer = load_breast_cancer() print(cancer.keys()) >>> dict_keys([‘data’, ‘target’, ‘frame’, ‘target_names’, ‘DESCR’, ‘feature_names’, ‘filename’]) print(cancer.target_names) print(cancer.feature_names) >>> [‘malignant’ ‘benign’] [‘mean radius’ ‘mean texture’ ‘mean perimeter’ ‘mean area’ ‘mean smoothness’ ‘mean compactness’ ‘mean concavity’ ‘mean concave points’ ‘mean symmetry’ ‘mean fractal dimension’ ‘radius error’ ‘texture error’ ‘perimeter error’ ‘area error’ ‘smoothness error’ ‘compactness error’ ‘concavity error’ ‘concave points error’ ‘symmetry error’ ‘fractal dimension error’ ‘worst radius’ ‘worst texture’ ‘worst perimeter’ ‘worst area’ ‘worst smoothness’ ‘worst compactness’ ‘worst concavity’ ‘worst concave points’ ‘worst symmetry’ ‘worst fractal dimension’]
breast_cacer데이터의 기본 구성 확인 후 train, test 데이터 분리
X_train, X_test, y_train, y_test = train_test_split(cancer.data, cancer.target, test_size=0.2, random_state=42 )
최적의 max_depth 찾기
from sklearn.model_selection import KFold cv = KFold(n_splits=5) # Desired number of Cross Validation folds #n_splits값이 클수록 오래걸림 accuracies = list() max_attributes = X_test.shape[1] depth_range = range(1, max_attributes) # Testing max_depths from 1 to max attributes # Uncomment prints for details about each Cross Validation pass for depth in depth_range: fold_accuracy = [] rand_clf = RandomForestClassifier(max_depth = depth) # print(“Current max depth: “, depth, ”
“) for train_fold, valid_fold in cv.split(train): f_train = train.loc[train_fold] # Extract train data with cv indices f_valid = train.loc[valid_fold] # Extract valid data with cv indices model = rand_clf.fit(X_train, y_train) valid_acc = model.score(X_test, y_test) fold_accuracy.append(valid_acc) avg = sum(fold_accuracy)/len(fold_accuracy) accuracies.append(avg) # print(“Accuracy per fold: “, fold_accuracy, ”
“) # print(“Average accuracy: “, avg) # print(”
“) # Just to show results conveniently df = pd.DataFrame({“Max Depth”: depth_range, “Average Accuracy”: accuracies}) df = df[[“Max Depth”, “Average Accuracy”]] print(df.to_string(index=False))
max_depth간에 accuracy 차이가 거의 없긴 하다. max_depth 6부터 정확도가 조금 떨어지므로 max_depth=5로 설정했다. 이제 랜덤포레스트 모델에 피팅을 시켜보자.
rand_clf = RandomForestClassifier(criterion=’entropy’, bootstrap=True, random_state=42, max_depth=5) rand_clf.fit(X_train, y_train) y_pred = rand_clf.predict(X_test) print(‘훈련세트 정확도: {:.3f}’ .format(rand_clf.score(X_train, y_train))) print(‘테스트세트 정확도: {:.3f}’ .format(rand_clf.score(X_test, y_test))) >>>훈련세트 정확도: 0.993 >>>테스트세트 정확도: 0.965
train set 정확도는 99.3%, test set 정확도는 96.5%로 아주 높다. 조금 의심스럽긴 하지만 잘 맞췄다고 할 수 있다.
from sklearn.metrics import confusion_matrix, classification_report print(confusion_matrix(y_test, y_pred)) print(classification_report(y_test, y_pred))
모델 훈련을 시켰으니 마지막으로 feature_importances를 확인해보자.
import seaborn as sns import numpy as np %matplotlib inline # feature importance 추출 print(“Feature importances:
{0}”.format(np.round(rand_clf.feature_importances_, 3))) # feature별 importance 매핑 for name, value in zip(cancer.feature_names , rand_clf.feature_importances_): print(‘{0} : {1:.3f}’.format(name, value)) # feature importance를 column 별로 시각화 하기 sns.barplot(x=rand_clf.feature_importances_ , y=cancer.feature_names)
worst area, worst concave points, mean concave points 세 개 변수의 중요도가 가장 높다고 할 수 있다.
[머신러닝] 랜덤 포레스트 (Random Forest) 개념 및 예제 실습 / 독버섯 예제
랜덤 포레트스
랜덤 포레스트 – Random Forest
집단 학습을 기반으로 고정밀 분류, 회귀, 클러스트링 구현
학습 데이터로 다수의 의사결정 트리를 만들고 그 결과의 다수결 결과 유도로 높은 정밀도
무작위 샘플링과 다수의 의사결정 트리 => Random Forest
결정 트리(Decision Tree)
분류(Classification)와 회귀(Regression) 모두 가능한 지도 학습 모델 중 하나
결정 트리는 스무고개 하듯이 예/아니오 질문을 이어가며 학습
한 번의 분기 때마다 변수 영역을 두 개로 구분
매, 펭귄, 돌고래, 곰을 구분한다고 생각해보자
Terminal Node는 LeafNode 라고도 함
프로세스
1. 데이터를 가장 잘 구분할 수 있는 질문을 기준으로 나누기
2. 나뉜 각 범주에서 또 다시 데이터를 가장 잘 구분할 수 있는 질문을 기준으로 나눔
* 이를 지나치게 많이 하면 아래와 같이 오버피팅
결정 트리에 아무 파라미터를 주지 않고 모델링하면 오버피팅
가지치기(Pruning) – 오버피팅 막기 전략
트리에 가지가 너무 많으면 오버피팅
한 노드가 분할하기 위한 최소 데이터수를 제한 옵션 : min_sample_split = 10
max_depth를 통해서 최대 깊이를 지정max_depth = 4이면, 깊이가 4보다 크게 가지를 치지 않음
알고리즘: 엔트로피(Entropy), 불순도(Impurity)
불순도(Impurity)
해당 범주 안에 서로 다른 데이터가 얼마나 섞여 있는지를 뜻함
한 범주에 하나의 데이터만 있다면 불순도가 최소(혹은 순도가 최대)
한 범주 안에 서로 다른 두 데이터가 정확히 반반 있다면 불순도가 최대(혹은 순도가 최소)
결정 트리는 불순도를 최소화(혹은 순도를 최대화)하는 방향으로 학습을 진행
엔트로피(Entropy)
불순도(Impurity)를 수치적으로 나타낸 척도
엔트로피가 높다는 것은 불순도도 높다는 뜻
엔트로피가 1이면 불순도가 최대
한 범주 안에 데이터가 정확히 반반 있다는 뜻
엔트로피 공식 (Pi = 범주 i에 속한 데이터의 비율)
엔트로피 예제
경사, 표면, 속도 제한을 기준으로 속도가 느린지 빠른지 분류해놓은 표
X 가 경사, 표면, 속도 제한
Y 가 속도(라벨)
경사 표면 속도제한 속도 steep bumpy yes slow steep smooth yes slow flat bumpy no fast steep smooth no fast
속도 라벨에는 slow, slow, fast, fast로 총 4개의 examples
Pi는 범주 i에 속한 데이터의 비율
i를 slow라고 했을 때, P_slow = 0.5 (2/4)
P_fast도 0.5 (ff/ssff)
전체 엔트로피 : 0.5 + 0.5 = 1
정보 획득 (Information gain)
엔트로피가 1인 상태에서 0.7인 상태로 바뀌었다면 정보 획득(information gain)은 0.3
분기 이전의 엔트로피에서 분기 이후의 엔트로피를 뺀 수치가 바로 정보 획득량
결정 트리 알고리즘은 information gain을 최대화하는 방향으로 학습이 진행
Information gain = entropy(parent) – [weighted average] entropy(children)
경사를 기준으로 첫 분기
{steep : [‘slow’, ‘slow’, ‘fast’], flat: ‘fast’ } – flat: 엔트로피 0 entropy(parent) = 1 entropy(flat) = 0 entropy(steep) = P_slow * log2(P_slow) – P_fast * log2(P_fast) entropy(steep) = – (2/3) * log2(2/3) – (1/3) * log2(1/3) entropy(steep) = 0.9184 [weighted average] entropy(children) = weighted average of steep * entropy(steep) + weighted average of flat * entropy(flat) entropy(children) = 3/4 * (0.9184) + 1/4 * (0) entropy(children) = 0.6888 # 최종 엔트로피
information gain = entropy(parent) – [weighted average] entropy(children)
information gain = 1 – 0.6888 = 0.3112
표면 기준 분기, 속도제한 기준 분기의 information gain 을 비교
가장 정보 획득이 많은 방향으로 학습 진행
랜덤 포레스트(Random Forest)
결정 트리 하나만으로도 머신러닝 가능
결정 트리의 단점은 훈련 데이터에 오버피팅이 되는 경향이 큼
여러 개의 결정 트리를 통해 랜덤 포레스트를 만들면 오버피팅 되는 단점을 해결
원리
건강의 위험도를 예측
건강의 위험도를 예측하기 위해서는 많은 요소를 고려
성별, 키, 몸무게, 지역, 운동량, 흡연유무, 음주 여부, 혈당, 근육량, 기초 대사량 등 수많은 요소가 필요
Feature가 30개라 했을 때 30개의 Feature를 기반으로 하나의 결정 트리를 만든다면 트리의 가지가 많아질 것이고, 이는 오버피팅의 결과를 야기
30개의 Feature 중 랜덤으로 5개의 Feature만 선택해서 하나의 결정 트리 생성
계속 반복하여 여러 개의 결정 트리 생성
여러 결정 트리들이 내린 예측 값들 중 가장 많이 나온 값을 최종 예측값으로 지정
이렇게 의견을 통합하거나 여러 가지 결과를 합치는 방식을 앙상블(Ensemble)이라고 함
하나의 거대한 (깊이가 깊은) 결정 트리를 만드는 것이 아니라 여러 개의 작은 결정 트리를 만드는 것
분류 : 여러 개의 작은 결정 트리가 예측한 값들 중 가장 많은 값
회귀 : 평균값
파라미터
n_estimators: 랜덤 포레스트 안의 결정 트리 갯수
n_estimators는 클수록 좋지만 그만큼 메모리와 훈련 시간이 증가
max_features: 무작위로 선택할 Feature의 개수, 일반적으로 Default
랜덤 포레스트 example
DataSet
UCI 머신러닝 레포지토리에 공개된 독버섯 관련된 데이터
https://archive.ics.uci.edu/ml/datasets/Mushroom
8,124종류의 버섯의 특징과 독이 있는지 적혀있는 데이터 세트
p,x,s,n,t,p,f,c,n,k,e,e,s,s,w,w,p,w,o,p,k,s,u
e,x,s,y,t,a,f,c,b,k,e,c,s,s,w,w,p,w,o,p,n,n,g
e,b,s,w,t,l,f,c,b,n,e,c,s,s,w,w,p,w,o,p,n,n,m
p,x,y,w,t,p,f,c,n,n,e,e,s,s,w,w,p,w,o,p,k,s,u
e,x,s,g,f,n,f,w,b,k,t,e,s,s,w,w,p,w,o,e,n,a,g
e,x,y,y,t,a,f,c,b,n,e,c,s,s,w,w,p,w,o,p,k,n,g
한 줄이 버섯 한 종류
첫 번째 열 독 유무 p: poisionous, e: edible
두 번째 열 버섯 머리 모양 b:벨, c:원뿔, x:볼록, f:평평, k:혹, s:오목
네 버째 열 버섯의 머리 색 n:갈색, b:황갈색, c:연한갈색, g:회색, r:녹색, p:분홍색, u:보라색, e:붉은색, w:흰색, y:노란색
나머지는 필요시 UCI 사이트에서 확인
↓ 랜덤포레스트 example
ML – RandomForest.html 0.30MB
[Python] Ensemble(앙상블)
이 게시글은 오로지 파이썬을 통한 실습만을 진행한다. 앙상블 기법중 RandomForest의 개념 및 원리를 알고자하면 아래 링크를 통해학습을 진행하면 된다.
https://todayisbetterthanyesterday.tistory.com/48?category=822147
실습에 사용할 데이터는 아래의 데이터이다. kaggle에서 제공하는 데이터이며, 상세한 내용은 아래와 같다.
otto_train.zip 1.69MB
id: 고유 아이디
feat_1 ~ feat_93: 설명변수
target: 타겟변수 (1~9)
RandomForest는 Bagging 기법에 학습 모델의 분산을 줄이기 위해서, 변수까지 특정 개수로 무작위 추출을 하는 방법으로 학습을 진행한다. RandomForest는 Tree기반의 앙상블 기법으로 sklearn을 통해 간단하게 구현이 가능하다. 그럼에도 불구하고, 보통 좋은 성능을 갖는다고 알려져있다.
이 RandomForest에 대해서 Python을 활용한 실습을 진행해보자.
1. 데이터 처리
# data 처리를 위한 library import os import pandas as pd import numpy as np from sklearn.model_selection import train_test_split
# 현재경로 확인 os.getcwd()
위의 코드는 파이썬 해당파일(.py / .ipynb)의 위치경로를 표기해주는 것이다. 만약 데이터가 다른 경로에 존재한다고 하였을 때, 전 경로를 확인하기 위해서 자주 사용한다.
# 데이터 불러오기 # kaggle data data = pd.read_csv(“./otto_train.csv”) data.head()
먼저 이 kaggle데이터는 target변수가 class_1~9까지 문자형식으로 되어있다. 그렇기에 이 문자형식의 target을 수치형 데이터(1~9)로 변환해줄 필요가 있다.
또한 이 kaggle데이터는 기업 및 단체가 데이터를 제공하기에 위와 같이 feature에 대한 정보를 알 수 없도록 해놓았다. 이를 통해 파이썬 실습을 진행할 것이다.
# shape확인 nCar = data.shape[0] # 데이터 개수 nVar = data.shape[1] # 변수 개수 print(‘nCar: %d’ % nCar, ‘nVar: %d’ % nVar )
otto_train 데이터에는 61878개의 행과 95가지의 변수가 존재한다. target을 제외하면 94가지 features가 존재하는 것이다. 먼저 target의 형변환을 해주어고 무의미한 변수를 제거해야한다.
# 무의미한 변수 제거 data= data.drop([‘id’],axis=1)
# 타겟 변수의 형변환 mapping_dict = {‘Class_1’ : 1, ‘Class_2’ : 2, ‘Class_3’ : 3, ‘Class_4’ : 4, ‘Class_5’ : 5, ‘Class_6’ : 6, ‘Class_7’ : 7, ‘Class_8’ : 8, ‘Class_9’ : 9,} after_mapping_target = data[‘target’].apply(lambda x : mapping_dict[x]) after_mapping_target
target의 형태가 “Class_1” – 1 ~ “Class_9” – 9 로 변환되었다. 이를 통해서 이제 classification을 진행할 것이다.
# features/target, train/test dataset 분리 feature_columns = list(data.columns.difference([‘target’])) X = data[feature_columns] y = after_mapping_target train_x, test_x, train_y, test_y = train_test_split(X, y, test_size = 0.2, random_state = 42) # 학습데이터와 평가데이터의 비율을 8:2 로 분할| print(train_x.shape, test_x.shape, train_y.shape, test_y.shape) # 데이터 개수 확인
2. Random Forest 적합
Random Forest
#기본적인 randomforest모형 from sklearn.ensemble import RandomForestClassifier from sklearn.metrics import accuracy_score # 정확도 함수 clf = RandomForestClassifier(n_estimators=20, max_depth=5,random_state=0) clf.fit(train_x,train_y) predict1 = clf.predict(test_x) print(accuracy_score(test_y,predict1))
위의 Random Forest는 트리의 깊이를 5로 제한하고 20개의 Sampling을 통해 학습을 진행한다. 그 결과 정확도가 59%정도 나왔다. 분류기로 사용하기에는 좋지않은 정확도이다.
Random Forest Sample개수 증가
# sample 100개, tree depth – 20 clf = RandomForestClassifier(n_estimators=100, max_depth=20,random_state=0) clf.fit(train_x,train_y) predict2 = clf.predict(test_x) print(accuracy_score(test_y,predict2))
위의 경우에는 sample개수를 100개로 늘렸다. 그랬더니 정확도가 대략 78%까지 증가하였다. 그렇다면 무한하게 많이 sample개수를 증가시키면 되지 않는가? 하는 생각이 들 수도 있다. 하지만, sample개수가 증가한다고 하여서 무작정 성능이 향상되지는 않는다. 아래 경우를 보자
# sample 300개, tree depth – 20 clf = RandomForestClassifier(n_estimators=300, max_depth=20,random_state=0) clf.fit(train_x,train_y) predict2 = clf.predict(test_x) print(accuracy_score(test_y,predict2))
위의 경우는 샘플 개수를 300개까지 늘린 것이다. 그랬더니 성능이 100개보다 좋지 않는 61% 정도로 떨어졌다. 이것은 ML의 전반적인 특성이기도 하다. 무작정 학습의 반복횟수/sample개수를 늘린다는 것이 성능의 향상을 장담하지는 않는다. 그렇기에 해당 데이터에 맞는 선정 모델의 parameter은 분석가가 경험적으로 찾거나, 아니면 해당 분야에서 통상적으로 쓰이는 parameter를 알아야한다.
그렇다면 위의 모델에서 최적의 n_estimators parameter가 100이라고 가정하고, 이번에는 트리의 깊이를 늘려보자.
Random Forest Tree 깊이 증가
# sample 100개, tree depth – 100(max) clf = RandomForestClassifier(n_estimators=100, max_depth=100,random_state=0) clf.fit(train_x,train_y) predict2 = clf.predict(test_x) print(accuracy_score(test_y,predict2))
위의 결과를 보면 sample개수가 100개이고, 깊이를 최대로 허용하였을때, 정확도가 대략 81%까지 상승하였다. 물론 RandomForest를 포함한 ensemble모형에는 수많은 parameter들이 존재하기에 다른 parameter의 최적값 또한 찾아낸다면 더 좋은 성능을 발휘할 것이다.
하지만 그렇게되면 설명을 해야할게 너무 많아지기에 아래 sklearn 링크를 통해서 알아보기를 추천한다.
https://scikit-learn.org/stable/modules/generated/sklearn.ensemble.RandomForestClassifier.html
So you have finished reading the 랜덤 포레스트 예제 topic article, if you find this article useful, please share it. Thank you very much. See more: 파이썬 랜덤포레스트 예제, 랜덤포레스트 활용, 랜덤포레스트 예측 파이썬, 랜덤포레스트 변수중요도, 랜덤포레스트 파이썬, 랜덤포레스트 class_weight, 랜덤포레스트 실습, 랜덤포레스트 회귀